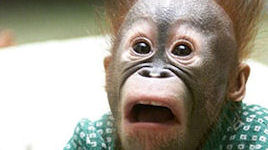
- Орангутаны научились использовать орудия с выгодой для себя [2019-03-22]
- Мозг собак отличил настоящие слова от тарабарщины [2019-03-22]
- Какаду Гоффина выклевали палочки из картонки и использовали их в быту [2019-03-22]
- Орангутанов уличили в создании крючков [2019-03-22]
- Любовь самок гуппи к ярким самцам объяснили генами и освещением [2019-03-22]
- Орангутаны рассказывают друг другу о прошлом [2019-03-22]
- Блеск и нищета этологии [2018-05-03]
- Почему хозяева похожи на своих собак? [2018-03-15]
- Интервью с Анатолием Протопоповым [2019-02-13]
- Цирки: развлечение vs мучение [2018-12-15]
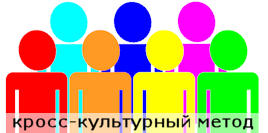
• 2.1. Generating and testing motion trajectories
• 2.2. An evolutionary task analysis of intention categories—Why bother moving?
• 2.4. Experiment 1: Six-category judgment task with German adults
• 2.4.4. Experiment 1 discussion
• 2.5. Experiment 2: four-category judgment task with German children
• 2.5.4. Experiment 2 discussion
• 2.6. Experiment 3: four-category judgment task with German adults
• 2.6.4. Experiment 3 discussion
• 2.7. Experiment 4: four-category task with Shuar adults
• 2.7.4. Experiment 4 discussion
• 3. Further analysis—cues and algorithms for categorization
1. Introduction
The movements of living things are a rich source of cues about their goals and intentions, and humans and other animals are exquisitely attuned to these cues. We can take into account an animal's species, age, sex, facial expression, body posture, and signaling behaviors when making such judgments, as well as the motion's ecological and social contexts. Often, though, whole-body motion alone is enough to trigger a strong impression of intentionality: A diving seagull seems intent on attack, and a circling dog intent on play. It is not even necessary to have an organism-shaped body in motion, as Heider and Simmel (1944) showed. Their animations of moving triangles and circles elicited strong subjective impressions of goals and intentions (e.g., of one triangle trying to catch another). Since their pioneering work, such simple, two-dimensional displays have been shown to produce intention-from-motion judgments across different non-Western cultures (Morris & Peng, 1994), across different ages as young as 9 months (Berry & Springer, 1993, Csibra et al., 1999), and across different social primate species (e.g., chimpanzees—Uller & Nichols, 2000). In recent years, the literature on perception of animate motion has grown enormously (for recent reviews, see Gelman & Opfer, 2002, Johnson, 2000, Rakison & Poulin-Dubois, 2001, Scholl & Tremoulet, 2000), including research on the specific cues that drive intention inferences and the development of such inferences in infancy (e.g., Csibra et al., 1999, Gergely et al., 1995, Opfer, 2003, Tremoulet & Feldman, 2000), intentionality inferences in nonhuman animals (Goto, Lea, & Dittrich, 2002), the brain regions underlying intention-from-motion judgments (Blakemore et al., 2003, Castelli et al., 2000), and their impairment (Castelli, Frith, Happé, & Frith, 2002). In addition, there have been many studies on non-whole-body motion cues, such as the motion of different body parts with respect to each other, beginning with the pioneering work of Johansson (1973) on biological motion perception using point-light displays (for a recent example of emotion perception from point-light displays, see Dittrich, Troscianko, Lea, & Morgan, 1996). This research is different from our whole-body motion approach in that it assumes that one can see greater detail about the moving body and that the mechanisms underlying judgments in the two settings are probably somewhat different.
It is important to investigate intention-from-motion judgments because making correct inferences about the imminent behavior of others has adaptive benefits, and misunderstanding what another individual intends to do can seriously impair survival or reproduction. For example, animals must accurately discriminate predatory pursuit from sexual courtship, and aggressive rivalry from benign play. Moreover, the faster we can make these judgments, the more time we have to respond appropriately, whether to approach a playing stranger, flee an angry brother, or follow a coaxing parent. Whole-body motion information (i.e., the path that a whole organism takes through its environment) can be especially useful in allowing faster decisions because such judgments can be made at a greater distance (before gait, body posture, or facial expression can be perceived) and when other information is hidden or degraded, as when chasing prey at dusk through thick forest. Evidence that human intentionality judgments are largely automatic (Scholl & Tremoulet, 2000) also suggests that selection has favored making them very quickly and accurately.
Although previous studies have demonstrated that certain motion patterns reliably elicit subjective impressions of intent, some important questions remain unanswered. First, how accurate are these processes of inferring intention from motion? Second, are these processes universals in the human cognitive architecture, or are they culturally specific? Other studies have demonstrated a human tendency to perceive intent in a few types of biologically significant motion (such as pursuit and evasion) and to describe them in appropriate terms (e.g., Abell, Happé, & Frith, 2000), but here, we want to measure the accuracy of these unconscious perceptual inferences and extend the range of intentions under consideration. We also want to examine the accuracy of intention-from-motion judgments across cultures, especially in a non-Western, nonindustrial society with minimal exposure to television, computers, video games, and other sources of artificial motion stimuli.
It is generally desirable to test hypotheses about human cognitive universals in many cultures. But here, we follow a slightly different logic for cross-cultural tests by selecting two cultures that are very distant in terms of the recency of shared cultural heritage and at far ends of the spectrum of technological complexity (German & Barrett, in press). Thus, although we use only two cultures—and therefore our conclusions about universality must remain tentative—by comparing two such disparate ones, we avoid the pseudoreplication problem inherent in cross-cultural studies that test college students of different nationalities, who nonetheless experience much shared environment. Moreover, the many possible sources of difference in performance between the two cultures that we investigate all work against our hypothesis.
In this paper, we (1) introduce a novel method for generating six types of naturalistic motion stimuli, each expressing a certain intention (chasing, courting, following, guarding, fighting, or playing); (2) report four experiments that test how well adults and children can categorize and discriminate between these expressed intentions, given only the motion stimuli; (3) examine whether accuracy in identifying particular kinds of intentional motion is culturally specific or universal, and (4) analyze the motion cues that allow these intention-from-motion judgments.
2. Method overview
2.1. Generating and testing motion trajectories
To see how well people can distinguish between different intentions based on motion cues alone, our research followed several steps. First, we used an evolutionary task analysis, that is, an analysis of the problems that lead organisms to move and to move in specific ways, to identify a set of basic animate intentions that would be useful for humans to distinguish and that seemed potentially discriminable given whole-body motion trajectories. Next, we recorded naturalistic examples of motion trajectories generated by people playing an interactive computer game, with incentives to achieve particular intentional goals. We then showed these recorded trajectories to adults in a multiple-choice categorization task and showed shorter versions to children and adults across cultures in a two-option forced choice task. Finally, we analyzed our participants' responses to assess their accuracy on intention-from-motion judgments, to determine which intentional categories are most easily confused and to uncover the specific motion cues used to infer intentions.
2.2. An evolutionary task analysis of intention categories—Why bother moving?
Animals move for a variety of reasons, only some of which have important adaptive consequences for others, and only some of which may be inferred from whole-body motion. In cases where motion can be used as a cue, the higher the fitness payoff for correctly identifying a particular intention, the greater the selective pressure would have been for the ability to discern that intention from whole-body motion. Thus, we began by considering which kinds of movement serve important adaptive functions of survival and reproduction across animal species (including humans). We restricted our attention to interactions between two individuals that could be observed from an overhead (third-person) perspective, as in most studies following Heider and Simmel (1944).
Moving has energetic costs; thus, animals are expected to move only when these costs are exceeded by some fitness benefits in the domains of survival and reproduction. Thus, the basic processes of natural and sexual selection may suffice to deduce a few paradigmatic goals of animate motion. Animals generally evolve to interact adaptively with various “fitness affordances” in their environments—things that are likely to affect the replication of their genes (Miller & Freyd, 1993, Miller & Todd, 1995). Positive fitness affordances, including resources such as food, shelter, and sexual partners, promote survival or reproduction. Negative fitness affordances, such as predators, pathogens, parasites, and sexual competitors, interfere with survival or reproduction. Animals evolve sensory-motor systems so they can approach the positives and avoid the negatives. If two animals offer mutually positive fitness yields, mutual approach usually results. In these cases, movement patterns represent solutions to simple positive-sum coordination games. When one animal is attracted to another animal, and this animal moves towards a mutually beneficial affordance, such as food or shelter, then leading and following can result, as when a litter of offspring trail behind a parent. If two animals threaten mutually negative yields, then mutual avoidance results.
The more complex cases of movement emerge given a zero-sum conflict, when one animal wants to be near the other, but the other wants to be far away. A deer is a positive (food) affordance for a tiger, but the tiger is a very negative (death) affordance for the deer. Such conflicts of interest lead to more extended and intricate interactions, transforming simple mutual approach into relentless pursuit, and simple avoidance into desperate evasion (Miller & Freyd, 1993). Likewise, unreciprocated sexual interest leads to sexual pursuit and evasion, as when male ducks sexually harass females (McKinney, Derrickson, & Mineau, 1983). When the zero-sum conflict concerns a spatially fixed resource (e.g., a good food patch, nest site, or herd of fertile females), the resource's current holder tends to guard it, and the would-be acquirer tries to invade the location in various ways. In these cases, movement patterns represent strategies in complex zero- or negative-sum dynamical games, which are analyzed in differential game theory using differential equations (e.g., Dockner et al., 2000, Isaacs, 1999), in evolutionary game theory using dynamic optimization methods (e.g., Cressman, 2003), or in artificial life research using coevolutionary simulations (e.g., Cliff & Miller, 1995, Cliff & Miller, 1996). Often, these pursuit–evasion games require the use of unpredictable “mixed strategies” or “protean behavior” to defeat the expectations of evolutionary opponents (Driver & Humphries, 1988, Miller, 1997).
This qualitative task analysis led us to six basic categories of intentional motion (Blythe, Todd, & Miller, 1999). In the survival domain, we selected (1) pursuit and evasion (abbreviated here as “chasing”) and (2) fighting, both of which may occur within species as social behavior or between species as predation or resource competition. In the reproductive domain, we chose (3) courting and being courted (abbreviated as “courting”), the richest interactive stage between mate search and copulation. Spanning both domains, we chose (4) leading and following (abbreviated as “following”), as in a parent leading an offspring to shelter (which increases the offspring's chance of survival and the mother's reproductive success), (5) guarding and invading (abbreviated as “guarding”), as in one individual guarding a food patch that another keeps trying to exploit, and (6) playing, which can be seen as “practicing” moves for other domains (Fagen, 1981, Steen & Owens, 2001). These six categories seem to account for a great deal of natural animate motion, especially motion with significant adaptive costs, benefits, and risks.
Previous work focused primarily on the first two categories, chasing and fighting (e.g., Dittrich & Lea, 1994). Here, by expanding the set of intentions under investigation, we hoped to get a broader understanding of intention-from-motion inferences.
2.3. Stimulus generation
Our next step was to gather a set of naturalistic whole-body motion trajectories that reflected these six types of intentions. To do so, we developed a two-person computer game played by adult German-speaking participants in Berlin, Germany. Two participants sat in separate rooms at computers. Each participant used a mouse to control a V-shaped arrowhead (red or black) on the computer screen, which was programmed to always point in the direction of current motion. Both participants could see both arrowheads, but the screen was otherwise empty. The arrowheads were not simply cursors that could be moved instantly by a mouse movement, as in normal PC operation. Rather, to simulate realistic physical dynamics, the mouse's current position relative to the mouse pad specified a screen position towards which the arrowhead was programmed to move from its current position, given simulated linear and angular momentum, limited linear and rotational rates of acceleration and deceleration, and a limited top speed. Thus, when a participant moved the mouse, the resulting motion was “smoothed” with more realistic acceleration dynamics, while maintaining the overall path of motion that the participant intended.
The participants were instructed to move their arrowheads with a particular intention in mind (as explained further below)—for example, Participant 1 should “pursue” Participant 2, while Participant 2 “evades” Participant 1. Each pair of participants played four trials for each of the six intention categories (in both roles) and knew what the six categories were before starting. The resulting two-player motion patterns in each 90-s trial were recorded, to be presented as the motion trajectories in subsequent experiments eliciting intention-from-motion judgments.
In earlier experiments (Blythe et al., 1999), we gave the two interacting participants explicit instructions about how to achieve each intentional goal; for instance, we said that chasing means moving quickly to intercept the other participant. However, we felt that such instructions might bias or limit the motion strategies that participants might adopt. For the current studies, we wanted a less directive way to elicit intuitive motion strategies and schemas. To do this, we gave each player just the label of the intentional motion that we wanted them to generate in each trial (e.g., chasing, invading) and told them to move in a way that would be distinctive and easily categorized by third-party observers. Then, to motivate players to produce trajectories that closely matched their intuitive motion schemes, we provided performance-contingent payoffs.
These payoffs were based on the accuracy with which a third participant (as a “judge”) could categorize the motion trajectories into the intention types. This judge sat in a separate room, watched the on-screen arrowheads moving about, and at the end of each 90-s trial, made a forced-choice categorization among the six possible intentions. Every time the judge selected the correct category (i.e., the category that the other two players had been instructed to generate), all three participants received immediate performance feedback and a small monetary reward (1 Deutschmark, worth about 50 US cents at the time of data collection in 1998). This method, borrowed from experimental economics, imposed minimal constraints on the motion strategies that could be used to exemplify each intention category, and maximized opportunities for learning in this three-player, positive-sum coordination game. In all, 10 sets of participants (each with two motion generators and one judge) produced 40 trajectories for each intention. From these, we eliminated ambiguous stimuli by selecting four examples of each intentional category that elicited the most accurate judgments in pretrial tests (which were the choices made by the original judges and by two additional sets of judges who saw either 10–50 or 10–90 s of a subset of the trajectories). These 24 most prototypical trajectories were the motion stimuli that we used in the categorization experiments described in the next sections. The distinctiveness of the trajectories representing different intentions is apparent in Fig. 1, where we show three-dimensional plots of the x–y position of both arrowheads as a function of time on the z axis. Additionally, Quicktime movie samples of the stimuli can be found at http://www.sscnet.ucla.edu/anthro/faculty/barrett/motion.htm.
2.4. Experiment 1: Six-category judgment task with German adults
2.4.1. Methods
To determine how accurately people can make intention-from-motion judgments, participants were shown the 24 prototypical trajectories (four examples for each of the six intentions) in randomized order, 6 as initial practice trials (1 from each category) and the remaining 18 as experimental trials. The trajectories each lasted 40 s. The first 10 and last 40 s of the original 90-s trials were removed to eliminate anomalies at the very beginning and very end of the trajectories. As participants watched each trajectory on a computer screen, they judged which intention was being shown and were free to change their judgments over the course of the trial by moving their mouse over a screen area indicating their current judgment. Early and accurate judgments were encouraged by paying participants according to the total proportion of time that they chose the correct category within each trial. Payment was received after the experiment was completed, and participants received no feedback during the course of the experiment. Although category choice could change dynamically over the course of viewing a trajectory, here, the participants' final category choices are analyzed, and these final category choices are used to calculate the hit and false alarm rates reported below. Of the 288 total trials, there were 4 in which participants failed to make a choice, and these have been removed from the analysis.
2.4.2. Participants
Participants were 12 native German-speaking adults in Berlin (6 males, 6 females). These were different participants than those who generated the motion trajectories and had no knowledge of how the trajectories were generated.
2.4.3. Results
Table 1 shows the rates of hits and false alarms for each motion category on this task, as well as the pattern of confusion of different categories. The average hit rate across participants and categories was 75%. Because participants could choose between six categories, chance performance was 1/6, or 17%. (In comparison, accuracy in our earlier studies using more directive verbal instructions and no performance-based payoffs was only 49%—see Blythe et al., 1999) The overall hit rate was well above chance (binomial test, one tailed, chance proportion=.17, p<.001). In addition, the individual hit rates for each of the six categories, shown in Table 1, were all well above chance (binomial test, one tailed, chance proportion=.17, p<.001). This result suggests that German adults can indeed make accurate judgments of intentions based on whole-body motion cues alone.
Table 1.
Confusions between judged and original intentions made by German adults (Experiment 1) |
![]() |
Selected category | ![]() | |||||||
---|---|---|---|---|---|---|---|---|---|
![]() |
True category | Chasing | Courting | Following | Guarding | Fighting | Playing | Totals | ![]() |
![]() |
Chasing | 42 | 0 | 1 | 1 | 1 | 3 | 48 | ![]() |
![]() |
Courting | 0 | 41 | 0 | 2 | 0 | 4 | 48 | ![]() |
![]() |
Following | 7 | 1 | 38 | 1 | 0 | 0 | 48 | ![]() |
![]() |
Guarding | 2 | 1 | 0 | 30 | 2 | 12 | 48 | ![]() |
![]() |
Fighting | 2 | 2 | 0 | 5 | 33 | 6 | 48 | ![]() |
![]() |
Playing | 6 | 1 | 1 | 2 | 6 | 31 | 48 | ![]() |
![]() |
Totals | 59 | 46 | 40 | 41 | 42 | 56 | 288 | ![]() |
![]() |
Hit rate (%) | 88 | 85 | 79 | 63 | 69 | 65 | 75 | ![]() |
![]() |
False alarm rate (%) | 7 | 2 | 1 | 5 | 4 | 10 | 5 | ![]() |
This table shows the number of times that a given intention was selected (across the top) for a trajectory that had been generated with a particular original “true” intention (down the left side). The main diagonal thus presents correct categorizations. Responses are pooled across participants. |
Comparison of hit rates for different categories shows that there were differences in how well different categories of motion were identified. The highest hit rates were for chasing (88%) and courting (85%), and the lowest hit rates were for guarding (63%) and playing (65%), although these were still well above chance. Difference of proportion tests (Rosenthal & Rosnow, 1991) were used to compare the proportion of hits for each category with the proportion of hits for the other five categories, pooled (i.e., the global hit rate, excluding the category under test). This analysis revealed that chasing had a significantly higher hit rate than did the other categories pooled (difference of proportions test, two tailed, p<.05); courting was marginally significantly higher (p<.1, two tailed), and guarding and playing had significantly lower hit rates (p<.05, two tailed).
However, because categories with similar hit rates were not significantly different from each other (e.g., chasing vs. courting), we grouped together the three categories with the highest hit rates, chasing, courting, and following (pooled hit rate 84%), and compared them with the grouped data from the lower hit rate categories, guarding, fighting, and playing (pooled hit rate 65%). Hit rates of the chasing/courting/following category were higher than that of the guarding/fighting/playing category (p<.001, difference of proportions test, two tailed).
Similar analyses were conducted with false alarm rates (see Table 1). Again, difference of proportion tests were used to compare the false alarm rate for each category with the global false alarm rate for the other five categories. Playing (10%) and chasing (7%) had significantly higher false alarm rates than did other categories pooled (p<.05 for both). Courting (2%) and following (1%) had significantly lower false alarm rates (p<.05), and the other two categories were not significant.
Using the same category groupings reported above, we found that false alarm rates to the chasing/courting/following category (mean 3%) were significantly lower than to the guarding/fighting/playing category (mean 6%; p<.01, difference of proportions test, two tailed). Thus, it appears that chasing, courting, and following collectively have higher accuracy and lower false alarm rates than do guarding, fighting, and playing.
2.4.4. Experiment 1 discussion
These results show that German adults are able to accurately infer intentions from motion, at a level well above chance, for all six categories we tested. The patterns of hits and false alarms are also suggestive. The hit rate, an index of success of categorization, is highest for chasing and significantly higher than the mean rate for the other five categories pooled. That participants should be good at distinguishing chasing is consistent with studies showing that a motion schema for chasing schema emerges early in infancy (Csibra, Bíró, Koós, & Gergely, 2003) and with the evolutionary importance of inferring intentions in the context of predator–prey interactions (Barrett, in press). Because of the possible costs of failing to identify a predation event, principles of error management (Haselton & Buss, 2000; and the “fire alarm principle” from Darwinian medicine, Nesse & Williams, 1995) suggest that false alarms to this category should be relatively high, as indeed they were (7%), second only to playing (10%). Interestingly, chasing, courting, and following all yielded high hit rates, despite the fact that they all involve approach of one agent towards another, suggesting that, despite this similarity, they are important categories to distinguish given the different intentions involved. However, the fact that some of these similar categories were sometimes confused (e.g., following was often mistaken for chasing) while some were not (chasing and courtship were never confused) deserves further investigation.
Although the false alarm rate to playing is high (although not significantly higher than that for chasing), the hit rate to playing is much lower than to chasing. The hit rate to playing is significantly lower than the pooled rate. Playing is an unusual category. Because it can serve as “practice” for other forms of interaction (Steen & Owens, 2001), it may contain elements of other motion types, leading to high rates of misses (i.e., a low hit rate), as well as false alarms. A similar argument applies to the occasional confusions between fighting and guarding/invading, which may also arise because they share common motion elements.
2.5. Experiment 2: four-category judgment task with German children
2.5.1. Methods
Given our results showing that adults can distinguish intention types fairly accurately based on motion cues, and past research showing that even young infants can reason intentionally about particular motions (Csibra et al., 1999), we wanted to test whether young children could also infer intentions accurately from our motion stimuli. To this end, a smaller, simpler set of the trajectories was constructed. These consisted of twelve 20-s segments (the first half of the 40-s trajectories from Experiment 1) of four basic intentions: chasing, following, fighting, and playing. These were displayed using circular dots. Participants were asked during each trajectory which of two possible intentions it represented (e.g., “Are the two dots chasing or playing?”). This two-option forced-choice method simplified the task by not requiring the children to bear in mind all four possible categories in each trial. Participants expressed their choice verbally, and it was noted by the experimenter. Participants were allowed up to one re-viewing of each trajectory, if requested.
Each participant saw four warm-up trials, in which they were asked to verbally describe the behavior of the dots, followed by 12 experimental choice trials. A computer algorithm was used to generate a randomized set of comparisons for each participant. Each participant saw three exemplars of each of the four motion categories, order randomized across all 12. For each exemplar, a choice between two categories was offered, which always included the correct category and one of the other three categories, order randomized. In most cases, participants saw all three possible comparisons for each category (the algorithm in some cases generated two versions of the same category pair for a given participant, but removal of these had no significant effect on the analyses below).
2.5.2. Participants
Participants were 36 German children, ages 3–5, recruited from local schools and daycare centers. There were eleven 3-year-olds (5 girls, 6 boys; age range 3.3–3.11; mean age=3.8), fourteen 4-year olds (5 girls, 9 boys; age range 4.1–4.11; mean age=4.5), and eleven 5-year-olds (5 girls, 6 boys; age range 5.1–5.9; mean age=5.4). All children were German speakers, and the experiment was administered by a native German-speaking research assistant using a portable laptop computer in a quiet room in the school.
2.5.3. Results
The data are summarized in Table 2, which shows the hit rates, and Table 3, which shows the false alarm rates. The overall accuracy in this task was 60%, where chance was 50%. The mean hit rates for each group were compared with this chance level using binomial tests. Three-year-olds, with a mean hit rate of 52%, were not significantly above chance. Both 4- and 5-year-olds were significantly above chance, with mean hit rates of 64% and 63%, respectively (p<.01, binomial test, one tailed).
Table 2.
Hit rates for Experiments 2–4 (four-category tasks) |
![]() |
Category | Experiment 2 | Experiment 3 | Experiment 4 | ![]() | |||
---|---|---|---|---|---|---|---|---|
![]() |
German 3-year-olds | German 4-year-olds | German 5-year-olds | German children pooled | German adults | Shuar adults | ![]() | |
![]() |
n | 11 | 14 | 11 | 36 | 40 | 23 | ![]() |
![]() |
Chasing (%) | 33 | 40 | 39 | 40 | 73 | 80 | ![]() |
![]() |
Fighting (%) | 67 | 76 | 64 | 69 | 83 | 71 | ![]() |
![]() |
Following (%) | 45 | 76 | 73 | 67 | 80 | 75 | ![]() |
![]() |
Playing (%) | 61 | 64 | 76 | 67 | 83 | 88 | ![]() |
![]() |
All categories pooled (%) | 51 | 64 | 63 | 60 | 80 | 79 | ![]() |
Hit rates were computed by dividing the number of times that a category was selected correctly by the number of times that a category of motion was shown. In all cases, participants are selected from among two possible choices. |
Table 3.
False alarm rates for Experiments 2–4 (four-category tasks) |
![]() |
Category | Experiment 2 | Experiment 3 | Experiment 4 | ![]() | |||
---|---|---|---|---|---|---|---|---|
![]() |
German 3-year-olds | German 4-year-olds | German 5-year-olds | German children pooled | German adults | Shuar adults | ![]() | |
![]() |
n | 11 | 14 | 11 | 36 | 40 | 23 | ![]() |
![]() |
Chasing (%) | 33 | 31 | 21 | 29 | 23 | 25 | ![]() |
![]() |
Fighting (%) | 64 | 36 | 27 | 41 | 15 | 12 | ![]() |
![]() |
Following (%) | 45 | 38 | 52 | 44 | 13 | 14 | ![]() |
![]() |
Playing (%) | 52 | 38 | 48 | 44 | 29 | 35 | ![]() |
![]() |
All categories pooled (%) | 48 | 36 | 37 | 39 | 20 | 21 | ![]() |
False alarm rates were computed by dividing the number of times that a category was selected incorrectly by the number of times that a category was available as an incorrect choice. In all cases, participants are selected from among two possible choices. |
To examine children's performance for different categories of motion, we compared hit and false alarm rates for different categories using difference of proportion tests, as in Experiment 1. As Table 2 shows, the hit rate patterns across the four categories were similar for 3-, 4-, and 5-year-olds. For all three age groups pooled, chasing had a significantly lower hit rate (40%) than did the other three categories pooled (68%; difference of proportions test, p<.05, two tailed). None of the other three categories was significantly different, suggesting a general consistency in the ability to identify motion categories, with the exception of chasing.
The overall rate of false alarms (see Table 3) was significantly higher for 3-year-olds (48%) than for 4- (36%) and 5-year-olds (37%), which were not significantly different (difference of proportions test, two tailed). The patterns of false alarm rates across categories were less consistent than for hit rates and changed with age. For the youngest children, the rate of false alarms to fighting (64%) was significantly higher than for the other three categories pooled (43%, p<.05), and the rate of false alarms to chasing (33%) was significantly lower than for the other three categories pooled (54%, p<.05). For 4-year olds, there were no significant differences, and by age 5, false alarms to following (52%) are significantly higher than to the other three categories (32%; p<.05), and false alarms to chasing are significantly lower (21% vs. 42%; p<.05). For all three age groups, chasing received the fewest false alarms.
2.5.4. Experiment 2 discussion
The results show that, on a four-category task, children are able to accurately infer the intentional category of a given motion trajectory at above-chance levels by age 4. Performance increased significantly from age 3 to 4, but not from age 4 to 5. Moreover, as Experiment 3 will show, children in the 3- to 5-year range perform at a level well below that of adults on the same task. The emergence of above-chance performance by age 4 may be partly influenced by theory of mind development at this age, as found in many previous studies of intentionality (Wellman, Cross, & Watson, 2001), but we think that it is more likely that the improved accuracy in children aged 4 and 5 reflects an improved ability to understand artificial visual displays or to understand our verbal labels for intentional actions, given that nonverbal techniques have shown that infants can attribute goals to moving objects (Csibra et al., 2003).
A surprising result of Experiment 2 was that hit rates for chasing were low, and false alarm rates to this category were low as well, suggesting that children avoided selecting this category. This stands in contrast to the results of Experiment 1, in which adults had both high hit and high false alarm rates for chasing. We suspect that this aspect of children's performance does not reflect a lack of understanding of pursuit and evasion; indeed, Csibra et al. (2003) have shown that even infants are able to attribute the goals of pursuit and evasion to moving dots. Rather, we suspect that we used a word to describe chasing, verfolgen, which many children of this age do not understand, and which some of the children asked us to define. Had we used the term jagen—which more strongly connotes predator–prey interaction, which children at this age understand well (Barrett, in press)—children may have shown more accuracy on this intention type.
2.6. Experiment 3: four-category judgment task with German adults
2.6.1. Methods
To check that the shorter, 20-s versions of our motion stimuli used in Experiment 2 allowed accurate intention judgments in principle, the same task was also run with a sample of German adults. Stimuli and methods were identical to Experiment 2, and the same algorithmically generated randomization pattern of motion comparisons was used.
2.6.2. Participants
Participants were 40 German adults recruited in the Berlin area. The sample consisted of 26 women and 14 men, with an age range of 18–37 years (mean age=24 years, S.D.=5).
2.6.3. Results
The hit and false alarm rates for German adults on the four-category task are shown in Table 2, Table 3. Overall accuracy was 80%, well above chance (binomial test, p<.01, one tailed). Adult performance was significantly higher than the performance for German 3- to 5-year-olds, pooled (difference of proportions test, p<.01, two tailed).
Unlike the six-category task, but parallel to the results for children on the same four-category task, the hit rate to chasing (73%) was significantly lower than for the other three categories pooled (82%; difference of proportions test, p<.05, two tailed). There were no significant differences between hit rates for the other categories.
The overall rate of false alarms for German adults (20%) was significantly lower than the rate for German children, pooled (39%; p<.01, two tailed). For individual categories, the false alarm rate to following (13%) was significantly lower than for the other three categories pooled (23%; p<.05, two tailed), and the false alarm rate to playing (29%) was significantly higher than for the other three categories pooled (17%; p<.05, two tailed).
2.6.4. Experiment 3 discussion
German adults performed well above chance on this task and significantly better than the German children did. This suggests that shortening the trajectories did not render the task overly difficult, yet adults still have an advantage over children. While the overall hit rate on this task was similar to that in Experiment 1, here, chasing showed a relatively low hit rate compared with its relatively high hit rate in Experiment 1. This suggests that children's low hit rate to chasing may not be entirely due to problems with the category label; however, in contrast to children on the same task, adults' false alarm rate to chasing was not particularly low. Consistent with Experiment 1, false alarms to playing were high. That other categories are often mistaken for playing is again consistent with the idea that playing contains elements of other types of intentional motion.
Although the intention-from-motion inferences of German adults were quite accurate, it is unclear whether this was due to an evolved adaptation or a set of culturally specific intention schemas. It is possible that, rather than relying on universal motion schemas that reliably develop as part of the human cognitive architecture, participants could have relied on learned, culture-specific motion schemas shared by the participants who generated the motion trajectories and those who judged them. Our experiment could be viewed as a communication task, in which the motion-generating participants had incentives to produce motion trajectories that could signal specific intentional categories to the motion-judging participants. After all, our Berlin participants were raised in a common cultural milieu, experiencing the same evening “Sandman” cartoons and Autobahn driving tactics. Perhaps, our motion-generating participants were able to provide culturally specific “hints” about intention categories through certain motion cues to our motion-judging participants. Indeed, game theory research shows that genetic evolution and cultural learning can be equally effective at finding “correlated equilibria” that solve multiplayer coordination games, as our participants played (Antoci et al., 2000, Crawford, 1991). To test whether the accurate intention judgments in the previous experiments could have appeared solely due to shared cultural motion stereotypes, we ran an identical version of the four-category experiment in a culture far removed from that of urban Berlin: the Shuar of Ecuador.
2.7. Experiment 4: four-category task with Shuar adults
2.7.1. Method
The methods and stimuli for this task were identical to those used in Experiments 3 and 4, with the following exceptions. The same algorithmically generated randomization pattern of motion comparisons used in Experiments 2 and 3 was used. Instructions and category labels were presented in the Shuar language (by the first author), and participants were tested in their homes using a portable laptop computer.
2.7.2. Participants
The Shuar are hunter-horticulturalists living in the Amazon basin of Ecuador. While many Shuar adults have some exposure to Western culture through visits to Ecuadorian towns and cities, their culture is still based on Shuar hunter-horticulturalist traditions, with Shuar as the primary language.
Participants were 23 adult Shuar participants (20 males, 3 females; age range 15–58 years; mean age=30 years, S.D.=11), all native Shuar speakers, from a village in Morona Santiago Province, Ecuador. While all of the participants had seen televisions and computers during visits to the city, they had virtually no experience interacting directly with computers, and many were surprised to see dots moving on the screen.
2.7.3. Results
The hit and false alarm rates are summarized in Table 2, Table 3. The overall hit rate for the Shuar adults was 79%, above chance (binomial test, p<.01, one tailed), and not significantly different from that of German adults on the same task (80%; difference of proportions test). Moreover, the distribution of hit rates across categories was not statistically different for Shuar and German adults by chi-square test (two tailed) nor by individual difference of proportion tests. For the Shuar, only the hit rate for playing (88%) differed from the rate for the other three categories pooled (75%), being significantly higher (difference of proportions test, p<.05, two tailed).
As Table 3 shows, the pattern of false alarms was strikingly similar for Shuar and German adults. As with hit rates, the pattern of distributions of false alarms across categories did not differ between Shuar and German adults, either by chi-square test or by difference of proportion comparisons for individual categories. Within Shuar participants, the false alarm rate for fighting (12%) was significantly lower than the mean rate for the other three categories pooled (25%; p<.05), and the false alarm rate for playing (35%) was significantly higher (21%; p<.05).
2.7.4. Experiment 4 discussion
Perhaps the most surprising aspect of these results is that, despite the unfamiliarity of Shuar participants with computer graphics, and the fact that the motion stimuli were generated by German adults thousands of miles away, the performance of the Shuar adults on the task was statistically indistinguishable from that of German adults. They were statistically identical not only in the absolute level of accuracy, but also in the pattern of responses across the motion categories. The similarity in the pattern of false alarms is particularly striking, with playing receiving the most false alarms (as well as the same proportion) in both populations, and chasing second. This also replicates the pattern found with the six-category task in Experiment 1. The remarkable similarity of the results in two very different populations suggests that universal mechanisms of perception and judgment may be at work. These results lend support to the hypothesis that the motion schemas for chasing, fighting, following, and playing are not culturally contingent but may develop reliably as evolved adaptations for inferring intentions from physical motion trajectories, although this conclusion awaits replication in additional cultures.
3. Further analysis—cues and algorithms for categorization
What specific motion cues allowed participants to categorize intentions accurately across these four experiments? We consider simple cues that can be computed readily from a motion trajectory. For example, in chasing and following trials, both agents are usually heading in about the same direction at any particular time, with one (chasing or following) behind the other (evading or leading). This suggests a useful cue-relative heading that helps to distinguish these two intention types from others, such as fighting or courting, in which agents often face each other.
At any point, each moving agent can be described in terms of position, velocity (change in position), heading, and vorticity (change in heading). However, in a two-agent interaction, the absolute position and heading of each agent matter less than their relative position and heading. Likewise, for some intention categories, absolute vorticities (average rates of changing direction across both agents) may be diagnostic, but for others, relative vorticities (one agent changing direction much more often than the other) may be more informative. Thus, our task analysis suggested that the following motion cues may be useful for categorizing our two-agent trajectories:
In Table 4, we list the mean values of these cues computed across the four example trajectories for each intentional category used in Experiment 1. In four of the six categories, the agents' two roles are distinguishable (e.g., chasing vs. being chased, courting vs. being courted, following vs. being followed, guarding vs. attacking), hence, values are shown separately for each role.
Table 4.
Cue values for each agent in each type of intentional category |
![]() |
Category | Velocity (pixels/s) | Vorticity (degrees/s) | Relative Distance (pixels) | Relative velocity (pixels/s) | Relative vorticity (pixels/s) | Relative heading (degrees) | Relative angle (degrees) | ![]() | |||||
---|---|---|---|---|---|---|---|---|---|---|---|---|---|---|
![]() |
M | (S.D.) | M | (S.D.) | M | (S.D.) | M | (S.D.) | M | (S.D.) | M | M | ![]() | |
![]() |
Chasing | 740 | (189) | 12.2 | (3.1) | 340 | (114) | 212 | (65) | 0.6 | (0.21) | 21 | 345 | ![]() |
![]() |
Chased | 722 | (221) | 14.7 | (2.4) | 340 | (114) | 212 | (65) | 0.6 | (0.21) | 21 | 187 | ![]() |
![]() |
Courting | 612 | (289) | 10.3 | (1.8) | 228 | (156) | 338 | (177) | 2.9 | (0.87) | 32 | 147 | ![]() |
![]() |
Courted | 239 | (84) | 4.2 | (1.3) | 228 | (156) | 338 | (177) | 2.9 | (0.87) | 32 | 340 | ![]() |
![]() |
Following | 137 | (43) | 3.6 | (0.3) | 149 | (82) | 69 | (26) | 0.1 | (0.05) | 355 | 21 | ![]() |
![]() |
Followed | 142 | (55) | 3.9 | (0.6) | 149 | (82) | 69 | (26) | 0.1 | (0.05) | 355 | 196 | ![]() |
![]() |
Guarding | 398 | (202) | 8.1 | (1) | 410 | (189) | 284 | (59) | 0.47 | (0.13) | 343 | 289 | ![]() |
![]() |
Attacking | 516 | (306) | 10.1 | (2.9) | 410 | (189) | 284 | (59) | 0.47 | (0.13) | 343 | 134 | ![]() |
![]() |
Fighting | 661 | (115) | 15.7 | (2.8) | 413 | (123) | 118 | (72) | 1.2 | (0.34) | 88 | 62 | ![]() |
![]() |
Playing | 519 | (169) | 9.9 | (2.2) | 275 | (204) | 254 | (72) | 2.5 | (1.4) | 166 | 204 | ![]() |
Means and standard deviations (except for last two circular statistics) are shown computed over the four trajectory instances in each category that were presented to adults. Cue values are averaged over the 40-s length of each trajectory. |
These cues show strong diagnosticity for judging intention categories. For instance, low mean velocities are associated with being courted, following, and being followed. High mean absolute vorticities are associated with evasion and fighting. High relative vorticities are associated with courting, being courted, and playing. In an earlier work, we found that a variety of cue-based categorization strategies, applied to these motion cues, can yield similar adult-level accuracy levels on this task (Blythe et al., 1999). These strategies included linear cue integration, nonlinear cue integration by a neural network, and a lexicographic rule that uses a minimal number of cues. Using any reasonable categorization strategy, the simple motion cues listed above suffice to categorize these six basic intentions with a high level of accuracy. However, further work is needed to identify which specific motion cues and intention categorization strategies are actually used by people.
4. Conclusions
Judging intention from motion is a central adaptive problem for mobile animals and a foundation of human social cognition. In these four studies, we have shown that adults across two disparate cultures are accurate at judging the intentions of others based solely on motion patterns and that children begin making accurate intention-from-motion judgments using linguistic labels by age 4. The categorization errors are understandable in terms of the various functional similarities across the six basic intention categories and in terms of the how these similarities are manifest in the patterns of objective motion cue values. By developing a novel interactive method for generating distinct motion trajectory exemplars for each intention type, we have found some initial evidence that people across very different cultures achieve similar accuracy levels in intention-from-motion judgments, possibly reflecting a common set of intention-from-motion inference schemas.
The work presented here can be extended in many ways to investigate the interface between animate motion perception and social attributions of intention. This could include studying how autism, schizophrenia, and other conditions that impair social cognition may affect intention-from-motion judgments (e.g., Abell et al., 2000), and using brain imaging methods to identify cortical areas responsible for mapping between the perceptual and social domains (e.g., Adolphs, 1999). More generally, our method of generating behavioral data by asking participants to play compelling, interactive games with clear incentives could be extended to other domains of perception, cognition, decision making, and social behavior. As we have demonstrated here in the domain of motion trajectories, this approach can help to overcome the often-lamented trade-off between ecological validity and stimulus quantifiability.
Our results will, we hope, serve as an example of how researchers can investigate the adaptive fit between evolved decision heuristics and the environment structures that they exploit to guide fast, accurate, adaptive behavior. This perspective has enabled us to uncover initial evidence for a possible set of universal human psychological adaptations that map environment structure—perceptual cue patterns associated with different categories of interactive movement—to rapid, accurate judgments of intention from motion.
References
Abell et al., 2000 1.Do triangles play tricks? Attribution of mental states to animated shapes in normal and abnormal development. . Journal of Cognitive Development. 2000;15:1–20.
Adolphs, 1999 2.Social cognition and the human brain. . Trends in Cognitive Sciences. 1999;3:469–479. CrossRef
Antoci et al., 2000 3.Evolutionary selection of correlation mechanisms for coordination games. . Interaction and Market Structure. 2000;484:225–234.
Barrett, in press 4.Barrett, H. C. (in press). Adaptations to predators and prey. In D. M. Buss (Ed.), Handbook of evolutionary psychology. New York: Wiley.
Berry & Springer, 1993 5.Structure, motion, and preschoolers' perception of social causality. . Ecological Psychology. 1993;5:273–283.
Blakemore et al., 2003 6.The detection of contingency and animacy from simple animations in the human brain. . Cerebral Cortex. 2003;13:837–844. MEDLINE | CrossRef
Blythe et al., 1999 7.How motion reveals intention: categorizing social interactions. . In: Gigerenzer G, Todd PM, the ABC Research Group editor. Simple heuristics that make us smart. New York: Oxford University Press; 1999;p. 257–286.
Castelli et al., 2000 8.Movement and mind: a functional imaging study of perception and interpretation of complex intentional movement patterns. . NeuroImage. 2000;12:314–325. MEDLINE | CrossRef
Castelli et al., 2002 9.Autism, Asperger syndrome and brain mechanisms for the attribution of mental states to animated shapes. . Brain. 2002;125:1839–1849. MEDLINE | CrossRef
Cliff & Miller, 1995 10.Tracking the Red Queen: methods for measuring co-evolutionary progress in open-ended simulations. . In: Moran F, Moreno A, Merelo JJ, Cachon P editor. Advances in artificial life: Proceedings of the Third European Conference on Artificial Life (ECAL95). Berlin: Springer; 1995;p. 200–218.
Cliff & Miller, 1996 11.Co-evolution of pursuit and evasion: II. Simulation methods and results. . In: Maes P, Mataric MJ, Meyer J-A, Pollack J, Wilson SW editor. From animals to animats: 4. Proceedings of the Fourth International Conference on Simulation of Adaptive Behavior. Cambridge, MA: MIT Press; 1996;p. 506–515.
Crawford, 1991 12.An evolutionary interpretation of Van Huyck, Battalio, and Beil experimental results on coordination. . Games and Economic Behavior. 1991;3(1):25–59.
Cressman, 2003 13. . Evolutionary dynamics and extensive form games. Cambridge, MA: MIT Press; 2003;.
Csibra et al., 1999 14.Goal attribution without agency cues: the perception of ‘pure reason’ in infancy. . Cognition. 1999;72:237–267. MEDLINE | CrossRef
Csibra et al., 2003 15.One-year-old infants use teleological representations of actions productively. . Cognitive Science. 2003;27:111–133.
Dittrich & Lea, 1994 16.Visual perception of intentional motion. . Perception. 1994;23:253–268. MEDLINE
Dittrich et al., 1996 17.Perception of emotion from dynamic light-point displays represented in dance. . Perception. 1996;25:727–738. MEDLINE
Dockner et al., 2000 18. . Differential games in economics and management science. Cambridge, UK: Cambridge University Press; 2000;.
Driver & Humphries, 1988 19. . Protean behavior: the biology of unpredictability. Oxford, UK: Oxford University Press; 1988;.
Fagen, 1981 20. . Animal play behavior. New York: Oxford University Press; 1981;.
Gelman & Opfer, 2002 21.Development of the animate–inanimate distinction. . In: Goswami U editors. Blackwell handbook of childhood cognitive development. Oxford, UK: Blackwell; 2002;p. 151–166.
Gergely et al., 1995 22.Taking the intentional stance at 12 months of age. . Cognition. 1995;56:165–193. MEDLINE | CrossRef
German & Barrett, in press 23.German, T. P., & Barrett, H. C. (in press). Functional fixedness in a technologically sparse culture. Psychological Science.
Goto et al., 2002 24.Discrimination of intentional and random motion paths by pigeons. . Animal Cognition. 2002;5:119–127. MEDLINE | CrossRef
Haselton & Buss, 2000 25.Error management theory: a new perspective on biases in cross-sex mind reading. . Journal of Personality and Social Psychology. 2000;78:81–91. MEDLINE | CrossRef
Heider & Simmel, 1944 26.An experimental study of apparent behavior. . American Journal of Psychology. 1944;57:243–259. CrossRef
Isaacs, 1999 27. . Differential games: a mathematical theory with applications to warfare and pursuit, control, and optimization. New York: Dover; 1999;.
Johansson, 1973 28.Visual perception of biological motion and a model for its analysis. . Perception and Psychophysics. 1973;14:201–211.
Johnson, 2000 29.The recognition of mentalistic agents in infancy. . Trends in Cognitive Science. 2000;4:22–28.
McKinney et al., 1983 30.Forced copulation in waterfowl. . Behaviour. 1983;86:250–294.
Miller, 1997 31.Protean primates: the evolution of adaptive unpredictability in competition and courtship. . In: Whiten A, Byrne RW editor. Machiavellian intelligence: II. Extensions and evaluations. Cambridge, UK: Cambridge University Press; 1997;p. 312–340.
Miller & Freyd, 1993 32.Miller, G. F., & Freyd, J. J. (1993). Dynamic mental representations of animate motion: the interplay among evolutionary, cognitive, and behavioral dynamics. Cognitive Science Research Paper CSRP-290, University of Sussex.
Miller & Todd, 1995 33.The role of mate choice in biocomputation: sexual selection as a process of search, optimization, and diversification. . In: Banzhaf W, Eeckman FH editor. Evolution and biocomputation: computational models of evolution. Berlin: Springer; 1995;p. 169–204.
Morris & Peng, 1994 34.Culture and cause: American and Chinese attributions for social and physical events. . Journal of Personality and Social Psychology. 1994;67:949–971. CrossRef
Nesse & Williams, 1995 35. . Why we get sick: the new science of Darwinian medicine. New York: Random House; 1995;.
Opfer, 2003 36.Identifying living and sentient kinds from dynamic information: the case of goal-directed versus aimless autonomous movement in conceptual change. . Cognition. 2003;86:97–122. MEDLINE | CrossRef
Rakison & Poulin-Dubois, 2001 37.Developmental origin of the animate–inanimate distinction. . Psychological Bulletin. 2001;127:209–228. MEDLINE | CrossRef
Rosenthal & Rosnow, 1991 38. . Essentials of behavioral research: methods and data analysis. 2nd ed.. New York: McGraw-Hill; 1991;.
Scholl & Tremoulet, 2000 39.Perceptual causality and animacy. . Trends in Cognitive Sciences. 2000;4:299–309. CrossRef
Steen & Owens, 2001 40.Evolution's pedagogy: An adaptationist model pretense and entertainment. . Journal of Cognition and Culture. 2001;1:289–321.
Tremoulet & Feldman, 2000 41.Perception of animacy from the motion of a single object. . Perception. 2000;29:943–951. MEDLINE | CrossRef
Uller & Nichols, 2000 42.Goal attribution in chimpanzees. . Cognition. 2000;76:B27–B34. MEDLINE | CrossRef
Wellman et al., 2001 43.Meta-analysis of theory-of-mind development: the truth about false belief. . Child Development. 2001;72(3):655–684. MEDLINE
a Department of Anthropology, University of California, Los Angeles, Box 951553, Haines Hall 341, Los Angeles, CA 90095-1553, United States
b Max Planck Institute for Human Development, Berlin, Germany
c University of New Mexico, NM, United States
d Myretsu, Melbourne, Australia
Corresponding author. Tel.: +1 310 267 4260.
PII: S1090-5138(04)00080-7
doi:10.1016/j.evolhumbehav.2004.08.015
© 2005 Elsevier Inc. All rights reserved.