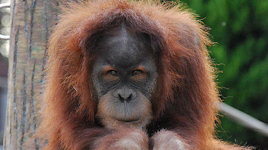
- Орангутаны научились использовать орудия с выгодой для себя [2019-03-22]
- Мозг собак отличил настоящие слова от тарабарщины [2019-03-22]
- Какаду Гоффина выклевали палочки из картонки и использовали их в быту [2019-03-22]
- Орангутанов уличили в создании крючков [2019-03-22]
- Любовь самок гуппи к ярким самцам объяснили генами и освещением [2019-03-22]
- Орангутаны рассказывают друг другу о прошлом [2019-03-22]
- Блеск и нищета этологии [2018-05-03]
- Почему хозяева похожи на своих собак? [2018-03-15]
- Интервью с Анатолием Протопоповым [2019-02-13]
- Цирки: развлечение vs мучение [2018-12-15]
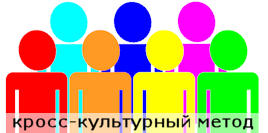
Real estate bookkeeping
5 Steps to successful real estate bookkeeping for investing.
www.bookstime.com
• 2. Are people vigilant against cheating even when cheating does not represent a loss? (Experiment 1)
• 2.2. Materials and procedure
• 3. Do people look for losses when cued to do so? (Experiment 2)
• 3.2. Materials and procedure
• 4. Are people biased against selecting the not-P and Q cards on a threat problem? (Experiment 3)
• 4.2. Materials and procedure
• 5.2. Materials and procedure
• 6.2. Materials and procedure
• 7. Were participants looking for nonconjunctive losses?
• 8.1. Were participants simply doing as instructed?
• 8.2. Were the tests logically biased against the UM account?
• 8.3. What role do subjective probabilities play in people's reasoning?
1. Introduction
According to evolutionary analyses of cooperation, the ability to detect and punish cheaters should be a design feature of adaptations for cooperation among nonkin (Trivers, 1971). There is, however, little consensus regarding the nature of the psychological mechanisms underlying cheater detection. In particular, it is not clear whether cheater detection is a function of utility calculations, as theories on utility maximization have proposed (e.g., Kirby, 1994, Manktelow & Over, 1991, Manktelow & Over, 1995, Oaksford & Chater, 1994), or of some more specialized mechanisms dedicated to the task of detecting cheaters (Cosmides, 1989, Cosmides & Tooby, 1989, Cosmides & Tooby, 1992). We review these rival accounts of cheater detection and provide experimental evidence that cheater detection is not merely a function of utility maximization but more specialized inference processes.
The prediction that people are competent at detecting cheating in cooperative situations was first tested by Cosmides (1989) using the Wason selection task, a standard test of conditional reasoning. In the selection task, participants are given a conditional rule of the form If P then Q and four cards bearing information related to the rule (P, not-P, Q, and not-Q), which they are to use when testing the truth of the rule or when testing compliance with it. Although originally devised to test people's reasoning about arbitrary propositions such as “If there is a D on one side of any card, then there is a three on its other side” (Wason, 1968), the task can also be used to study people's reasoning about social contract rules such as “If you receive Medicare, then you must be at least 65 years old” (i.e., rules regulating access to rationed benefits that form the basis of cooperative norms). Cosmides (1989) observed that people are much better at detecting violations of social contract rules, which constitute cheating, than they are at detecting violations of arbitrary conditional propositions.
Although people appear to be competent at detecting cheaters, there is considerable disagreement over the nature of the psychological mechanisms underlying cheater detection. While some evolutionary psychologists have proposed that cheater detection is a specific design feature of evolved psychological mechanisms for social cooperation (Cosmides, 1989, Cosmides & Tooby, 1989, Cosmides & Tooby, 1992, Cummins, 1996, Cummins, 1999, Hiraishi & Hasegawa, 2001), a widely favored alternate account of cheater detection maintains that it is simply the outcome of a desire to maximize one's utility (Kirby, 1994, Manktelow & Over, 1991, Manktelow & Over, 1995, Oaksford & Chater, 1994). We will refer to these two alternatives as design feature (DF) and utility maximization (UM), respectively.
In principle, there are numerous ways to maximize utility. The most plausible mechanism would be for people to maximize their gains while minimizing their losses. Yet, as an empirical matter, participants in selection task studies appear to be focused primarily on losses while ignoring gains. The experimental record has been summarized by Manktelow and Over (1995, p. 107), as follows:
“In all deontic selection tasks so far studied, the cards correctly chosen are ones which could show that the role or perspective of the subject was suffering a cost. That is, in this role or perspective, one prefers one's current state of affairs to the outcome that might be revealed by the cards. One has to have some ability to make decisions which could reveal serious costs to avoid the risk of suffering them continually.”
As a result, Manktelow and Over (1995) have suggested that losses may loom larger than gains in people's deontic reasoning (reasoning about obligations and entitlements that includes not only reasoning about social contracts but also reasoning about safety rules), as has also been observed in people's decision making in other domains (Kahneman & Tversky, 1979).
Whether this generalization holds is questionable. There are a substantial number of cheater-detection problems in which the person looking for cheaters is a third party with no immediate stake in the outcome and yet high levels of performance are elicited (e.g., Cosmides, 1989; Namka problems and Kaluame personal exchange). Such third parties do not stand to increase their personal utility, making performance on these versions of the task difficult for UM to explain; however, third-party punishment is an important component of some evolutionary accounts of cooperation (e.g., Boyd et al., 2003, Fehr & Fischbacher, 2004, Fehr et al., 2002, Gintis et al., 2003), suggesting that third-party cheater detection may also be an important DF of psychological adaptations for cooperation.
Despite such difficulties, there is a serious confound with previous selection task studies investigating people's reasoning about social contracts. In many cases, cheating constituted a loss to some party. Hence, there exists a much simpler and more parsimonious explanation of participants' performance on these tasks than the proposal that they evoke evolved cheater-detection mechanisms. Participants may be predisposed to preventing losses, which, coincidentally, are confounded with cheating.
Cheating is not invariably associated with a loss. Reciprocity typically involves the exchange of benefits; however, in order for both parties to benefit from the interaction, they must differ in their valuations of the items of exchange. This permits considerable leeway for individual differences in valuations put on the items of exchange such that one party might even view his or her own contribution to the exchange as a burden that he or she would just as soon be rid of. In situations such as these where one is trading away a personal burden, one could conceivably be better off in terms of immediate personal utility by being cheated as opposed to having the deal fall through (i.e., mutual noncooperation). In the experiments that follow, we employ such burden exchanges as means of disentangling cheating from losses.
Bluffing on threats is another type of social interaction in which the person making the threat could violate an implicit exchange with no loss to the person threatened. A person issuing a threat asserts that he or she will injure one unless one acts as demanded: “If you do X, then I will harm you.” A threat is like a cooperative exchange in which the person making the threat has first lowered the baseline utility of the individual being threatened [i.e., “I'm going to harm you (baseline lowered), but if you do X for me, then I will leave you be”]. If the person issuing the threat were bluffing, it would be analogous to acting “altruistically” (i.e., giving the benefit without demanding that the requirement is met) and would represent the best possible outcome from the perspective of the person who has been threatened.
Bluffing is not purely altruistic, though. Not only has the person issuing the threat lowered one's baseline utility, but in making the threat without the intention to enforce it, he or she stands to illicitly benefit at the expense of the threatened party should that person comply and not call the bluff. Knowing whether or not a person bluffs is strategically valuable information for future interactions—one can potentially avoid complying with empty threats. Bluff detection is similar to detection of prospective altruism. Brown and Moore (2000) define prospective–altruist detection as “the reliable detection of genuinely altruistic intentions before you enter a cooperative venture [which, unlike cheater detection,] excludes interaction with cheaters before exploitation” (pp. 26–27); hence, they view insincere altruism as a form of subtle cheating. The main difference between bluffing and insincere altruism is that insincere altruists exploit the false perception that they are kind, while bluffers exploit the false perception that they are not. With these provisos, we will assume that cheater detection and bluff detection evoke a common set of mental mechanisms. Therefore, in the experiments that follow, we also employ threat problems as a means of disentangling “cheating” from losses.
2. Are people vigilant against cheating even when cheating does not represent a loss? (Experiment 1)
In this experiment, we employed three different versions of the Wason selection task: a standard social exchange version, a burden exchange version, and a bluff-detection version. The latter two scenarios were designed such that cheating/bluffing and losses were unconfounded. We experimentally verified this by having participants rank the possible outcomes in terms of how bad they would be, where the worst possible outcome would constitute a loss. DF predicts that performance will track cheating/bluffing, whereas UM predicts that performance will track the worst possible outcomes (i.e., losses).
2.1. Participants
Twenty-seven people participated in this experiment, but three were later eliminated because they indicated that they were familiar with the Wason selection task. This left 12 males and 12 females who ranged in age from 20 to 38 years (mean=25.5 years). The participants were primarily students recruited at the Free University (Berlin, Germany). All participants were German speakers. Participants were paid for their participation in this experiment, which was conducted in German at the Max Planck Institute for Human Development (Berlin, Germany).
2.2. Materials and procedure
Participants first received a page of general instructions and three Wason selection tasks in counterbalanced order. Next, they received a page of instructions explaining the preference-ranking task and three preference-ranking problems based on and in the same order as the selection tasks. The three selection tasks were Gigerenzer and Hug's (1992) day-off problem, the refinery (burden exchange) problem in which a burden is unloaded in a social exchange (the complete scenario is available online at www-abc.mpib-berlin.mpg.de/users/fiddick/Fidd_Ruth_EHB.mht), and the Rocky (threat) problem (see also www-abc.mpib-berlin.mpg.de/users/fiddick/Fidd_Ruth_EHB.mht). The preference-ranking tasks presented scenarios from each selection task and asked participants to rank the four possible outcomes.
For a prototypical social exchange scenario, we employed Gigerenzer and Hug's (1992) day-off problem, which was written from the perspective of an employee checking to see whether his or her employer ever cheats on the rule “If an employee works on the weekend, then he gets a day off during the week.” In this scenario, being cheated is also the worst possible outcome. The refinery selection task employed a scenario in which a costly burden — refineries from a state-owned oil company — was being sold to foreign investors at a negligible price. The proposed deal was “If a foreign investor takes over a refinery, then he must pay the government 1000 DM.” The problem was designed so that failure to complete the sale (mutual noncooperation) would be the worst possible outcome from the state's point of view. Finally, the Rocky problem described a situation in which one is trying to figure out whether Rocky, a motorcycle gang member, ever bluffs when he makes threats. Rocky made the threat “If you touch my motorcycle, I'll break your legs,” and so he is bluffing when someone touches his motorcycle and yet he does nothing. This, however, is predicted to be ranked as the best possible outcome.
The preference-ranking tasks presented the selection task scenarios minus the cards and their description, and asked participants to rank the four possible outcomes (e.g., Rocky did nothing when the person touched his bike) according to how good or bad they were. They were told to rank the situations from 1 to 4 (1=best possible situation; 4=worst possible situation) using each number only once. Three preference-ranking tasks followed in an order matching the corresponding selection tasks.
2.3. Results and discussion
2.3.1. Preference rankings
The results of the preference-ranking and selection tasks are displayed in Table 1. The results of the preference-ranking tasks were as predicted. A majority of participants judged the employer's cheating to be the worst outcome for the day-off scenario (22 of 24, mean rank=3.9, where 4=worst possible outcome). By contrast, a majority of participants judged mutual noncooperation to be the worst outcome for the refinery scenario (mutual noncooperation: 16 of 24, mean rank=3.5; opponent cheats: 7 of 24, mean rank=2.7). Significantly more participants ranked mutual noncooperation as a worse outcome than being cheated [p<.05, sign test; p (success)=.5]. Still, a sizable minority ranked investor cheats as the worst possible outcome, suggesting a possible bimodal pattern of responses on the selection task. With respect to the Rocky scenario, a majority of participants judged double crossing (Rocky broke the person's legs even though the person left his bike alone), not bluffing, to be the worst outcome (double cross: 21 of 24, mean rank=3.8; bluff: 1 of 24, mean rank=1.54). Significantly more participants ranked double crossing as a worse outcome than bluffing [p<.0001, sign test; p (success)=.5].
Table 1.
Experiment 1: card selections and preference rankings |
![]() |
Outcome | Selection taska | Ranking taskb | ![]() |
---|---|---|---|---|
![]() |
Day-off scenario | ![]() | ||
![]() |
Mutual cooperation: Works on weekend and Gets day off | 8.3 | 1.96 (2) | ![]() |
![]() |
Employer cheats: Works on weekend and Does not get day off | 45.8c | 3.92 (4) | ![]() |
![]() |
Worker cheats: Does not work on weekend and Gets day off | 4.2 | 1.67 (1) | ![]() |
![]() |
Mutual noncooperation: Does not work on weekend and Does not get day off | 0 | 2.46 (2.5) | ![]() |
![]() |
Refinery scenario | ![]() | ||
![]() |
Mutual cooperation: Takes over refinery and Pays 1000 DM | 12.5 | 1.29 (1) | ![]() |
![]() |
Investor cheats: Takes over refinery and Does not pay 1000 DM | 62.5 | 2.71 (2) | ![]() |
![]() |
State cheats: Does not take over refinery and Pays 1000 DM | 0 | 2.46 (3) | ![]() |
![]() |
Mutual noncooperation: Does not take over refinery and Does not pay 1000 DM | 0c | 3.54 (4) | ![]() |
![]() |
Rocky scenario | ![]() | ||
![]() |
Threat Enforced: Touched motorcyle and Broken legs | 4.2 | 2.83 (3) | ![]() |
![]() |
Bluff: Touched motorcycle and Did not break legs | 41.7 | 1.54 (1) | ![]() |
![]() |
Double cross: Did not touch motorcycle and Broke legs | 0c | 3.83 (4) | ![]() |
![]() |
Compliance with threat: Did not touch motorcycle and Did not break legs | 0 | 1.79 (2) | ![]() |
The Ps and Qs follow the conventional mapping from a generic conditional If P then Q. |
a
Percentage of participants making card selection. bMean (median) ranking: 1=best possible outcome, 4=worst possible outcome. cUM predicts that these will be modal selections. |
2.3.2. Card selections
Performance on the selection tasks failed to support UM, but was consistent with DF (see Table 1; the complete pattern of responses for this and all other experiments can be found at www-abc.mpib-berlin.mpg.de/users/fiddick/Fidd_Ruth_EHB.mht). On the refinery problem, 63% of the participants selected the cards corresponding to the investors cheating. Contrary to UM, not a single participant selected the cards corresponding to mutual noncooperation, even though mutual noncooperation was judged as the worst possible outcome by 67% of the participants. The number of participants selecting the opponent cheats' cards and also judging opponent cheats as the worst possible outcome (4 of 15) was not significantly greater than chance (p>.20), while the number judging mutual noncooperation as the worst possible outcome (10 of 15) was significantly greater than chance (p<.01).
On the Rocky problem, there was a bimodal response pattern. Equal numbers of participants (42% each) selected the cards corresponding to bluffing or the Has touched Rocky's motorcycle card alone. Contrary to UM, no participant selected the cards corresponding to a double cross, even though a double cross was judged as the worst possible outcome by 87.5% of participants.
Consistent with both DF and UM, 46% of the participants selected the cards corresponding to the employer cheating on the day-off problem.
The results of this experiment support the DF prediction that people will look for cheaters even when they stand to benefit by being cheated. Contrary to UM, people's perception of relative losses did not determine the cards they selected.
3. Do people look for losses when cued to do so? (Experiment 2)
One potential difficulty in interpreting the results of Experiment 1 is that participants were specifically instructed to look for particular outcomes. We decided to repeat the contrasting scenarios from Experiment 1 with a loss-detection condition. The predictions remain the same for UM, whereas DF predicts that performance will decrease in the loss-avoidance condition since the instructions are at odds with the most relevant adaptive problem.
3.1. Participants
An additional 48 people participated in this experiment, but three were eliminated because they were familiar with the Wason selection task. This left 18 males and 27 females, who ranged in age from 20 to 40 years old (mean=24.5 years). The participants were primarily students and staff recruited at the Free University. Participants were paid for their participation.
3.2. Materials and procedure
Participants either received problems with cheater-detection/bluff-detection instructions, as in Experiment 1 (standard; n=22), or with loss-detection instructions (loss; n=23). The day-off problem was dropped since it does not discriminate between the two alternatives. Slight changes were made to the Rocky and refinery problems to prevent any biases towards bluff detection and cheater detection on the loss-detection versions of the problems (the complete scenarios are available at www-abc.mpib-berlin.mpg.de/users/fiddick/Fidd_Ruth_EHB.mht).
Where the original version of the Rocky problem featured the passage, “Perhaps he is only bluffing. The only way to find out if he is bluffing is to catch him in the act,” this was shortened to “Perhaps he is only bluffing” on both versions of the problem used here to eliminate this prompt to look for bluffing. Additionally, the instructions for the loss-detection version were changed to “Mark only those card(s) that you definitely need to turn over in order to see if Rocky's actions caused you to suffer a loss.”
The cheater-detection version of the refinery problem remained unchanged, while on the loss-detection version, the prompt to look for cheating (“You are curious to find out how honest these investors are, so you decide to investigate whether any of them has cheated the government”) was changed to “You are curious to find out how profitable business is with these investors, so you decide to investigate the present business transactions.” Additionally, the instructions for the loss-detection version were changed to “Mark only those card(s) that you definitely need to turn over in order to see if the actions of any of these foreign investors has caused you to suffer a loss.”
3.3. Results and discussion
The pattern of participants' card selections is provided in Table 2. While a substantial number of participants selected the cards corresponding to investor cheats and bluffing when explicitly instructed to do so, the levels of cheater detection and bluff detection dropped markedly with loss-detection instructions (refinery problem: 45.5% vs. 26.1%, Fisher's Exact Test, p>.10; Rocky problem: 36.4% vs. 8.7%, Fisher's Exact Test, p<.05).
Table 2.
Experiment 2: standard versus loss-detection instructions, pattern of card selections, and percentage of participants making each selection |
![]() |
Outcome | Standard (n=22) | Loss (n=23) | ![]() |
---|---|---|---|---|
![]() |
Refinery scenario | ![]() | ||
![]() |
Mutual cooperation: Takes over refinery and Pays 1000 DM | 9.1 | 4.3 | ![]() |
![]() |
Investor cheats: Takes over refinery and Does not pay 1000 DM | 45.5 | 26.1 | ![]() |
![]() |
State cheats: Does not take over refinery and Pays 1000 DM | 0 | 0 | ![]() |
![]() |
Mutual noncooperation: Does not take over refinery and Does not pay 1000 DM | 0a | 8.7a | ![]() |
![]() |
Rocky scenario | ![]() | ||
![]() |
Threat enforced: Touched motorcycle and Broke legs | 4.5 | 4.3 | ![]() |
![]() |
Bluff: Touched motorcycle and Did not break legs | 36.4 | 8.7 | ![]() |
![]() |
Double cross: Did not touch motorcycle and Broke legs | 0a | 4.3a | ![]() |
![]() |
Compliance with threat: Did not touch motorcycle and Did not break legs | 0 | 0 | ![]() |
The Ps and Qs follow the conventional mapping from a generic conditional If P then Q. |
a
UM predicts that these will be modal selections. |
Contrary to the predictions of UM, the levels of mutual noncooperation and double-cross selections were low in the loss condition. Although there were trends in the direction predicted by UM, they were not significant (refinery: Fisher's Exact Test, p>.20; Rocky: Fisher's Exact Test, p>.50).
Modal selections in the loss-detection condition were the selection of the No one paid 1000 DM card alone (refinery) and the Touched motorcycle card alone (Rocky); hence, one could argue that participants failed to select the Does not take over refinery and Broke legs cards because it is already obvious that the person has suffered a loss and, thus, one does not need to turn over the card. However, this line of explanation is unlikely, since this was also the modal selection pattern on the Rocky problem with standard instructions (50%, standard vs. loss, Fisher's Exact Test, p>.10). Indeed, the trend was for participants to select the Touched motorcycle card alone less often when they were explicitly instructed to look for losses (see also Section 7 on nonconjunctive losses).
4. Are people biased against selecting the not-P and Q cards on a threat problem? (Experiment 3)
Another alternative explanation is that participants may have had a default bias to reason logically, which would have entailed the selection of the P and not-Q cards (the predicted DF selection pattern in each case). Numerous studies have shown that participants will select the “illogical” not-P and Q cards on social contract versions of the selection task when it makes adaptive sense to do so (e.g., Cosmides, 1989, Fiddick et al., 2000, Gigerenzer & Hug, 1992, Holyoak & Cheng, 1995, Manktelow & Over, 1991, Politzer & Nguyen-Xuan, 1992), so this counterargument will not work for social contracts. However, the situation is less clear with respect to threats. In this experiment, we sought to investigate whether people will “illogically” select the not-P and Q cards on a threat problem when it is appropriate to do so.
4.1. Participants
An additional 45 participants recruited by e-mail from the University of London participated in this experiment. There were 19 males and 26 females who ranged in age from 18 to 50 years old (mean=23.3 years). They were primarily University of London students who were paid for their participation. The experiment was conducted in a seminar room at the University College London.
4.2. Materials and procedure
The procedure was the same as that employed in previous experiments, except that: (a) there was no preference-ranking task; (b) only the Rocky scenario was employed; (c) the statement “Perhaps he is only bluffing. The only way to find out if he is bluffing is to catch him in the act” was replaced by “Rocky is a tough and mean guy, so he never bluffs when he makes a threat. He's so mean that he might not keep his word and double-cross you instead. The only way to find out is to catch him in the act;” and (d) the instructions were changed to “Indicate only those card(s) that you would definitely need to turn over in order to determine whether or not Rocky has double-crossed any of these people” (the complete scenario is available at www-abc.mpib-berlin.mpg.de/users/fiddick/Fidd_Ruth_EHB.mht).
4.3. Results and discussion
The pattern of participants' selections is given in Table 3. Selection of the not-P and Q cards was the modal response [p<.000001, sign test; p (success)=.0625; i.e., 1 of 16 possible selection patterns]. The next most frequent selection pattern was P and not-Q (27%), suggesting that there may indeed be a bit of a bias towards logical responding. Nevertheless, even if participants in previous experiments were biased towards producing the logically correct selection, the existence of such a bias has difficulty explaining why only one participant in Experiment 2, and none in Experiment 1, selected the not-P and Q cards given that “not-P and Q” was the modal selection pattern in this experiment.
Table 3.
Experiment 3: Rocky problem (double-cross version), pattern of card selections, and percentage of participants making each selection (n=45) |
![]() |
Card pattern | Selection (%) | ![]() |
---|---|---|---|
![]() |
Threat enforced (P and Q) | 0.0 | ![]() |
![]() |
Bluff (P and not-Q) | 26.7 | ![]() |
![]() |
Double cross (not-P and Q) | 31.1a | ![]() |
![]() |
Compliance with threat (not-P and not-Q) | 0.0 | ![]() |
a
This is the predicted selection pattern if participants correctly identify potential double crosses. |
One further objection that could be raised is that our scenarios do not manipulate relevant utilities. Both the refinery problem and the Rocky problem involve third parties who might not stand to lose in the manner we have suggested.
5. Are people capable of detecting losses when they are directly involved in exchange? (Experiment 4)
Finally, it could be objected that participants' UM was not properly engaged by the materials because they were cued to adopt the perspective of third parties. However, if this were a factor, then it would also be reflected in and, thereby controlled for with, the preference rankings obtained in Experiment 1. Nevertheless, to rule out this confound, we devised a scenario in which the protagonist is personally involved in an exchange. Moreover, although a social exchange is described, no explicit conditional rule is mentioned, so there is no reason to suspect any logical bias in participants' responses.
5.1. Participants
An additional 56 participants from the University of London with no prior exposure to the selection task were recruited and paid as above. There were 19 males and 37 females who ranged in age from 18 to 39 years old (mean=21.2 years).
5.2. Materials and procedure
The procedure employed in this experiment was that employed in Experiment 2, with the exception that participants only solved one selection task and a corresponding preference-ranking task. The selection task described the exchange of a costly burden (the complete scenarios are available at www-abc.mpib-berlin.mpg.de/users/fiddick/Fidd_Ruth_EHB.mht). It told of a South American potato farmer, Juan, who had to dispose of some rotting potatoes and who subsequently struck a deal with some pig farmers exchanging quinoa (a grain) for his rotten potatoes. No explicit conditional rule was mentioned. There were two versions of the task. In the cheater-detection version, participants were instructed to check whether the pig farmers cheated. In the loss-detection version, participants were instructed to see whether Juan had suffered a loss. A corresponding preference-ranking task immediately followed. We predicted that participants would rank mutual noncooperation as the worst possible outcome.
5.3. Results and discussion
The results of the selection and preference-ranking tasks are presented in Table 4, listed under the headings cheater detection and loss detection. As predicted, a majority of participants judged mutual noncooperation to be the worst possible outcome in both the cheater-detection (mean=3.43, median=4, where 4=worst possible outcome) and loss-detection (mean=3.46, median=4) conditions. In the cheater-detection condition, significantly more participants judged mutual noncooperation to be a worse outcome than pig farmer cheats [PFC; 16 vs. 9, respectively, p<.05, sign test; p (success)=.5], whereas in the loss-detection condition, the difference in rankings failed to reach significance [15 mutual noncooperation vs. 13 PFC, p>.10, sign test; p (success)=.5]. Given these preference rankings, UM predicts a bimodal pattern of performance on the selection task, with roughly equal numbers of participants selecting the cards corresponding to mutual noncooperation and PFC.
Table 4.
Experiment 4: burden exchange with personal involvement, card selections, and preference rankings |
![]() |
Outcome | Selection taska | Ranking taskb | ![]() |
---|---|---|---|---|
![]() |
Cheater detection (n=28) | ![]() | ||
![]() |
Mutual cooperation: Juan gives rotten potatoes and Juan gets quinoa | 3.6 | 1.54 (1) | ![]() |
![]() |
PFC: Juan gives rotten potatoes and Juan gets nothing | 71.4 | 2.71 (2.5) | ![]() |
![]() |
Juan cheats: Juan gives nothing and Juan gets quinoa | 0 | 2.32 (3) | ![]() |
![]() |
Mutual noncooperation: Juan gives nothing and Juan gets nothing | 0c | 3.43 (4) | ![]() |
![]() |
Loss detection (n=28) | ![]() | ||
![]() |
Mutual cooperation: Juan gives rotten potatoes and Juan gets quinoa | 0 | 1.39 (1) | ![]() |
![]() |
PFC: Juan gives rotten potatoes and Juan gets nothing | 75 | 3.11 (3) | ![]() |
![]() |
Juan cheats: Juan gives nothing and Juan gets quinoa | 0 | 2.04 (2) | ![]() |
![]() |
Mutual noncooperation: Juan gives nothing and Juan gets nothing | 0c | 3.46 (4) | ![]() |
![]() |
Replication (n=25) | ![]() | ||
![]() |
Mutual cooperation: Juan gives rotten potatoes and Juan gets quinoa | 4 | 1.16 (1) | ![]() |
![]() |
PFC: Juan gives rotten potatoes and Juan gets nothing | 44 | 2.18 (2) | ![]() |
![]() |
Juan cheats: Juan gives nothing and Juan gets quinoa | 0 | 2.82 (3) | ![]() |
![]() |
Mutual noncooperation: Juan gives nothing and Juan gets nothing | 4c | 3.84 (4) | ![]() |
P=Juan gives rotten potatoes; Q=Juan gets quinoa; not-P=Juan gives nothing; not-Q=Juan gets nothing. |
a
Percentage of participants making card selection. bMean (median) ranking: 1=best possible outcome, 4=worst possible outcome. cUM predicts that these will be modal selections. |
Despite the fact that a majority of participants in both the cheater-detection and loss-detection conditions ranked mutual noncooperation as the worst possible outcome, not a single participant selected the cards corresponding to mutual noncooperation. One participant in the loss condition did, however, select the Juan gave this villager potatoes, Juan gave this villager nothing, and This villager gave Juan nothing cards — a selection pattern that was consistent with his ranking of PFC, followed by mutual noncooperation, as the worst possible outcomes.
Contrary to both UM and DF, the cards corresponding to PFC were selected by a majority of participants in both conditions. In the cheater-detection condition, only 6 of 20 participants selecting the PFC cards had also ranked PFC as the worst outcome, which is not significantly greater than chance [p>.15, sign test; p (success)=.25]. In the loss-detection condition, 11 of 21 participants selecting the PFC cards also judged PFC to be the worst outcome, which is significantly greater than chance [p<.01, sign test; p (success)=.25]. However, among the participants selecting the PFC cards, the proportion judging PFC to be the worst outcome in the loss-detection condition was barely different from the proportion (10 of 21) judging mutual noncooperation to be the worst possible outcome. In every case in which a participant's outcome rankings matched his or her card selections, PFC was ranked as the worst possible outcome. Given that no participants ranking mutual noncooperation as the worst possible outcome selected the matching cards, the matches that were observed may have been entirely coincidental.
The experiment produced two apparently anomalous results. First, the preference rankings were slightly at odds across the two conditions even though the basics of the scenario were identical. Second, the levels of cheater-detection selections were actually higher given loss-detection instructions. We believe that these anomalous results are not unrelated. Given that participants were cued to adopt the perspective of someone more directly involved in the exchange, the cheater-detection mechanism may have been more active than it was in previous experiments. Heightened activation of the cheater-detection mechanism may have biased participants to reinterpret “losses” in the loss-detection version of the selection task in terms of cheating affecting the subsequent preference-ranking task.
This explanation is easily tested by reversing the order of the tasks so that participants make their preference rankings before their cheater-detection mechanism is engaged by the selection task. Not only should a more consistent pattern of preference-ranking result but also the levels of cheater detection could actually decrease since prior performance of the preference-ranking task would reinforce the standard interpretation of “losses” such that losses and cheating are less likely to be confused.
6. Did prior solution of the selection task cause participants to reinterpret loss as cheating? (Replication)
6.1. Participants
An additional 26 participants from the University of London who had no prior exposure to the selection task were recruited and paid as above. One participant was eliminated from the study for improperly completing the ranking task. This left 11 males and 14 females who ranged from 18 to 30 years old (mean=21.3 years).
6.2. Materials and procedure
The materials and procedure were identical to those employed in Experiment 4, except that: (a) only the loss-detection condition was administered; (b) the order of the selection task and the preference-ranking task was reversed (the preference-ranking task now came first); and (c) minor adjustments to the wording and placement of the instructions were made solely to accommodate the altered order of the tasks.
6.3. Results and discussion
The results were as we predicted (see Table 4, replication). In contrast with the loss-detection condition in Experiment 4, significantly more participants ranked mutual noncooperation (n=23) as a worse outcome than PFC [n=2, p<.0001, sign test; p (success)=.5]. On the selection task, the level of PFC selections (44%) was significantly lower than that observed on the loss-detection condition in Experiment 4 (Fisher's Exact Test, p<.05). Most importantly, only two participants' card selections matched their preference rankings — significantly fewer matches than observed in the loss-detection condition (Fisher's Exact Test, p<.05). This decrease in the level of matching was largely driven by two factors: (a) only two participants ranked PFC as the worst outcome, and (b) fewer participants selected the PFC cards, reducing the odds of any spurious matching in accord with our interpretation of the results in Experiment 4.
7. Were participants looking for nonconjunctive losses?
We have assumed that people's decisions are cast in terms of conjunctive outcomes (e.g., someone took the benefit AND did not pay the cost), but participants in our studies may have instead been concerned with simple nonconjunctive losses (e.g., Rocky broke the person's legs, regardless of whether or not the person touched Rocky's motorcycle). If participants were focused on nonconjunctive losses, then UM predicts a different pattern of card selections. For example, in the Rocky problems, participants should select the Touched motorcycle and Did not touch motorcycle cards to see if Rocky broke the person's legs (i.e., the P and not-P cards; we would like to thank an anonymous reviewer for bringing these alternate predictions to our attention). We reanalyzed the results from all preceding experiments and in no instance did a significant number of participants select a pattern of cards corresponding to a search for nonconjunctive losses.
8. General discussion
In previous investigations of cheater detection on the selection task, cheating has been confounded with losses, making it difficult to determine whether people are specifically looking for cheaters or are seeking to maximize utility by looking for losses. However, the results reported here suggest that cheater detection is not guided by the search for losses, thereby lending support to proposals that cheater detection is a distinct DF of psychological adaptations for cooperative interactions.
8.1. Were participants simply doing as instructed?
One might be tempted to argue that the results are most parsimoniously explained by participants doing what they are instructed to do: when instructed to look for cheaters, they do so; when instructed to look for losses, they do not look for cheaters. What this asymmetric explanation ignores is that participants instructed to look for losses do not look for losses. UM theorists could, of course, argue that our loss-detection instructions were, in some way, flawed, but why should loss detection, as opposed to cheater detection, be so sensitive to the wording of the instructions? Participants not explicitly instructed to look for cheaters, as in Experiments 2 and 4, still continue to do so in significant numbers, even though the instructions to look for losses are at odds with looking for cheaters. Instead, the results suggest that people have a default tendency to look for cheaters as opposed to losses.
8.2. Were the tests logically biased against the UM account?
One might also be tempted to argue that cheater detection and bluff detection in these experiments were confounded with a content-independent logical solution to the task, namely, cheating and bluffing map onto logical violations of conditionals. Hence, people perform better on the cheater-detection and bluff-detection versions because of the participants' tendency to reason logically. This argument faces several difficulties. First, participants do not have a default tendency to solve the selection task logically, even when explicitly instructed to look for violations of the rule (Cosmides, 1989). Second, as discussed in relation to Experiment 3, people routinely make illogical selections when it is adaptively appropriate to do so. Typically, this has involved the search for cheaters on tasks employing “switched” social contracts (e.g., Cosmides, 1989) or “switched perspective” social contracts (e.g., Gigerenzer & Hug, 1992), but the same propensity to make illogical selections was also demonstrated for a threat scenario in Experiment 3. Finally, in Experiment 4, no conditional rules were employed, making the logic argument moot. Even if one were to argue that the situation described in Experiment 4 involved an implicit conditional, the exchange involved bilateral cheating options — either Juan or the pig farmers could cheat (see Gigerenzer & Hug, 1992) — so it is difficult to say whether the cheating in question represented a logical violation (P and not-Q) of an implicit conditional If P then Q or an illogical violation (not-P and Q).
8.3. What role do subjective probabilities play in people's reasoning?
The experiments reported here have focused on perceived utilities, ignoring subjective probabilities even though they play a prominent role in the many UM studies of the selection task (e.g., Kirby, 1994, Manktelow et al., 1995, Oaksford & Chater, 1994). It is people's subjective expected utility that people strive to maximize according to UM. What are we to make of these studies that purportedly demonstrate that cheater detection is sensitive to the probabilities of different outcomes? To begin with, there is nothing about the adaptive problem of social cooperation that precludes perceived probabilities from influencing the activation of the cheater-detection mechanism. However, there are also confounds that make these results difficult to interpret. Kirby's (1994), for example, attempted to demonstrate that subjective probabilities influence people's ability to detect violations of the rule “If a person is drinking beer, then the person must be over 21 years of age” by manipulating the age of the potential violators (e.g., 19-year-olds vs. 4-year-olds). Kirby argued that the younger is the person, the less likely that he or she would be violating the rule. However, it is also possible that 4-year-olds do not consider beer a benefit and, thus, are not likely to be cheaters. Similar difficulties plague the study of Manktelow et al. (1995): participants may be influenced by their probability estimates or may have simply adopted an alternative interpretation of response options.
9. Conclusion
Both UM and DF stress the role of perceived utilities in people's reasoning about social contracts, but utilities play different roles in the two approaches. For UM, the perception of utilities evokes decision-making processes aimed at maximizing utility by minimizing losses in particular. For DF, utilities play a more specific role in people's reasoning; they define, in part, instances of cheating. The results presented here suggest that perceived utilities play this more specific role assigned to them by DF and support the proposal that cheater detection is a distinct DF of psychological adaptations for cooperation, not the coincidental outcome of a more general desire to avoid losses.
Acknowledgments
This paper benefited greatly from comments by and discussions with Clark Barrett, Leda Cosmides, Jonathan Evans, Gerd Gigerenzer, David Over, Russ Revlin, John Tooby, and two anonymous reviewers of this paper. We would like to thank Jörn Schultz and Tanja Edelhauser for their help in translating the problems in Experiments 1 and 2 into German and in conducting the experiments. Initial drafts of this paper were written while Laurence Fiddick was a senior research fellow at the ESRC Center for Economic Learning and Social Evolution (ELSE) at the Department of Economics, University College London. We would like to thank ELSE and the ESRC for their support and generous funding for Experiments 3 and 4.
References
Boyd et al., 2003 1.The evolution of altruistic punishment. . Proceedings of the National Academy of Sciences of the United States of America. 2003;100:3531–3535. MEDLINE | CrossRef
Brown & Moore, 2000 2.Is prospective altruist-detection an evolved solution to the adaptive problem of subtle cheating in cooperative ventures? Supportive evidence using the Wason selection task. . Evolution and Human Behavior. 2000;21:25–37.
Cosmides, 1989 3.The logic of social exchange: Has natural selection shaped how humans reason? Studies with the Wason selection task. . Cognition. 1989;31:187–276. MEDLINE | CrossRef
Cosmides & Tooby, 1989 4.Evolutionary psychology and the generation of culture: Part II. Case study: A computational theory of social exchange. . Ethology and Sociobiology. 1989;10:51–97.
Cosmides & Tooby, 1992 5.Cognitive adaptations for social exchange. . In: Barkow J, Cosmides L, Tooby J editor. The adapted mind. New York, NY: Oxford University Press; 1992;p. 163–228.
Cummins, 1996 6.Evidence for the innateness of deontic reasoning. . Mind & Language. 1996;11:160–190.
Cummins, 1999 7.Cheater detection is modified by social rank: The impact of dominance on the evolution of cognitive functions. . Evolution and Human Behavior. 1999;20:229–248.
Fehr & Fischbacher, 2004 8.Third-party punishment and social norms. . Evolution and Human Behavior. 2004;25:63–87.
Fehr et al., 2002 9.Strong reciprocity, human cooperation and the enforcement of social norms. . Human Nature. 2002;13:1–25.
Fiddick et al., 2000 10.No interpretation without representation: The role of domain-specific representations and inferences in the Wason selection task. . Cognition. 2000;77:1–79. MEDLINE | CrossRef
Gigerenzer & Hug, 1992 11.Domain specific reasoning: Social contracts, cheating, and perspective change. . Cognition. 1992;43:127–171. MEDLINE | CrossRef
Gintis et al., 2003 12.Explaining altruistic behavior in humans. . Evolution and Human Behavior. 2003;24:153–172.
Hiraishi & Hasegawa, 2001 13.Sharing-rule and detection of free-riders in cooperative groups: Evolutionarily important deontic reasoning in the Wason selection task. . Thinking and Reasoning. 2001;7:255–294.
Holyoak & Cheng, 1995 14.Pragmatic reasoning with a point of view. . Thinking and Reasoning. 1995;1:289–313.
Kahneman & Tversky, 1979 15.Prospect theory analysis of decision under risk. . Econometrica. 1979;47:263–291.
Kirby, 1994 16.Probabilities and utilities of fictional outcomes in Wason's four-card selection task. . Cognition. 1994;51:1–28. MEDLINE | CrossRef
Manktelow & Over, 1991 17.Social roles and utilities in reasoning with deontic conditionals. . Cognition. 1991;39:85–105. MEDLINE | CrossRef
Manktelow & Over, 1995 18.Deontic reasoning. . In: Newstead S, Evans JStBT editor. Perspectives on thinking and reasoning: Essays in honour of Peter Wason. Hove, England: Lawrence Erlbaum; 1995;p. 91–114.
Manktelow et al., 1995 19.Probabilistic factors in deontic reasoning. . Thinking and Reasoning. 1995;1:201–220.
Oaksford & Chater, 1994 20.A rational analysis of the selection task as optimal data selection. . Psychological Review. 1994;101:608–631. CrossRef
Politzer & Nguyen-Xuan, 1992 21.Reasoning about promises and warnings: Darwinian algorithms, mental models, relevance judgments or pragmatic schemas?. . Quarterly Journal of Experimental Psychology. 1992;44A:401–421.
Trivers, 1971 22.The evolution of reciprocal altruism. . Quarterly Review of Biology. 1971;46:35–57. CrossRef
Wason, 1968 23.Reasoning about a rule. . Quarterly Journal of Experimental Psychology. 1968;20:273–281. CrossRef
a Center for Adaptive Behavior and Cognition, Max Planck Institute for Human Development, Berlin, Germany
b School of Psychology, James Cook University, Townsville, Australia
c Department of Psychology, McMaster University, Hamilton, Ontario, Canada
Corresponding author. School of Psychology, James Cook University, Townsville 4811, Australia. Tel.: +61 7 4781 4972; fax: +61 7 4781 5117.
PII: S1090-5138(06)00032-8
doi:10.1016/j.evolhumbehav.2006.05.001
© 2006 Elsevier Inc. All rights reserved.