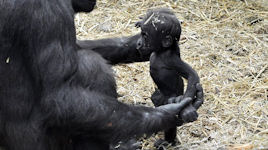
- Îðàíãóòàíû íàó÷èëèñü èñïîëüçîâàòü îðóäèÿ ñ âûãîäîé äëÿ ñåáÿ [2019-03-22]
- Ìîçã ñîáàê îòëè÷èë íàñòîÿùèå ñëîâà îò òàðàáàðùèíû [2019-03-22]
- Êàêàäó Ãîôôèíà âûêëåâàëè ïàëî÷êè èç êàðòîíêè è èñïîëüçîâàëè èõ â áûòó [2019-03-22]
- Îðàíãóòàíîâ óëè÷èëè â ñîçäàíèè êðþ÷êîâ [2019-03-22]
- Ëþáîâü ñàìîê ãóïïè ê ÿðêèì ñàìöàì îáúÿñíèëè ãåíàìè è îñâåùåíèåì [2019-03-22]
- Îðàíãóòàíû ðàññêàçûâàþò äðóã äðóãó î ïðîøëîì [2019-03-22]
- Áëåñê è íèùåòà ýòîëîãèè [2018-05-03]
- Ïî÷åìó õîçÿåâà ïîõîæè íà ñâîèõ ñîáàê? [2018-03-15]
- Èíòåðâüþ ñ Àíàòîëèåì Ïðîòîïîïîâûì [2019-02-13]
- Öèðêè: ðàçâëå÷åíèå vs ìó÷åíèå [2018-12-15]
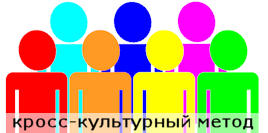
• 3. The empirics of frequency dependence
• 3.1. Individual decisions under fixed and sampled social groups
• 3.2. Only conformity produces internally homogeneous groups
• 3.3. Asch's study as an illustrative example
• Appendix A. Supplementary material
1. Introduction
Social scientists have long recognized the importance of frequency-dependent social learning (Asch, 1956, Bowles, 2004, Boyd & Richerson, 1985, Boyd & Richerson, 2005, Henrich & McElreath, 2003, Lumsden & Wilson, 1980, Richerson & Boyd, 2005, Sherif & Murphy, 1936). Frequency-dependent social learning postulates that individuals adopt a given behavior with a probability that varies in response to how common the behavior is in a relevant social group. Conformity is a type of frequency dependence that has received considerable attention. As formally defined (Boyd & Richerson, 1982, Boyd & Richerson, 1985), conformity is based on the following proposition. In a simple case with two behaviors R and B, where rt is the frequency of R in the population, conformity means that, in the near future, an individual exhibits behavior R with a probability less than rt if rt<1/2, but with a probability greater than rt if rt>1/2. In other words, individuals do not simply follow the majority; rather, they show a disproportionate tendency to follow the majority. They overrespond, so to speak, to frequency information. This feature of conformity is crucial because, as we show below, it homogenizes behavior within social groups. Other types of frequency dependence do not have this effect.
In spite of conformity's acknowledged importance, previous empirical research cannot identify conformity as a disproportionate tendency to follow the majority. Classic research in social psychology (Aronson et al., 2002, Asch, 1955, Asch, 1956), neuroscience experiments (Berns et al., 2005) in the tradition of Asch, and recent experiments with chimpanzees (Whiten, Horner, & de Waal, 2005) show that a focal individual is more likely to adopt a behavior as that behavior becomes more common. A simple model of nonconformity, however, makes exactly the same prediction, as do other hypotheses about positive social influences.
The distinctions, however, among different forms of positive influence are fundamental and not simply matters of definition. Below we present a general model of frequency dependence that includes both conformity and nonconformity as special cases. Although individual psychology is different for conformity and nonconformity, in both cases, the probability that a focal individual adopts a given behavior increases with the frequency of the behavior in the social group. Even so, dynamics at the group level are radically different. Conformity produces multiple steady states and can lead otherwise similar societies to evolve in completely different ways in the wake of small random effects (Bowles, 2004, Efferson & Richerson, 2007). Whatever behavioral variation may exist between groups, however, conformity produces social groups that are internally homogeneous in terms of behavior. Nonconformity, in contrast, increases behavioral variation within groups and decreases variation between groups (Efferson & Richerson, 2007). Whatever names we choose to attach to these forms of social influence, such distinctions are important. To demonstrate conformity as a force that homogenizes behavior within social groups, it is not enough to show simply that individuals adopt common behaviors. Researchers must also show that this inclination is disproportionate in the way described above. In this paper, we present a jointly theoretical and experimental approach to this problem.
Apart from its intrinsic interest, conformity has figured prominently in various discussions in the behavioral and evolutionary social sciences. Theoretically, conformity can be a valuable way to make good decisions in temporally and spatially variable environments (Henrich & Boyd, 1998). Imagine that R and B are two existing technologies. Individuals would like to choose the optimal technology, but returns are stochastic. The environment also varies in space and time, and so identifying the optimal technology is not easy. Assume that individuals experiment from time to time and learn individually as a result, and this produces a slight bias toward the optimal technology. As we show below, conformity exaggerates such a bias by filtering out a lot of the noise at the individual level. A powerful signal pointing toward the optimal technology is the result. By itself, however, conformity implies nothing about the optimality of individual decisions. It only exaggerates existing biases.
In addition to decision making, conformity has the interesting theoretical property that it reduces behavioral variation within populations while potentially increasing variation among populations. All else being equal, this increases the strength of selective pressures at the group level. Thus, in conjunction with the punishment of norm violations and the imitation of success, conformity plays a critical role in the study of how prosocial tendencies could have evolved in humans via cultural group selection (Boyd et al., 2003, Boyd & Richerson, 1982, Fehr & Fischbacher, 2003, Fehr & Fischbacher, 2004, Fehr & Gaechter, 2002, Guererk et al., 2006, Guzmán et al., 2006, Henrich, 2004, Henrich & Boyd, 2001). Conformity also appears to be critical in explaining aggregate patterns that characterize the diffusion of technological innovations (Henrich, 2001, Rogers, 1995).
2. How conformity works
Theoretically, conformity can be a valuable way to make decisions under uncertainty. Importantly, however, conformity is neither good nor bad by itself. It merely exaggerates existing biases in individual decision making. To illustrate, assume that technology R is optimal. Consider a group of N individuals, each of whom chooses R in a given period with probability rt. The probability that a majority of the individuals in the social group chooses the optimal technology when N is odd is simply,

Fig. 1 shows how P(majority opt) varies as a function of rt for four different values of N.
Importantly, P(majority opt)<rt if rt<0.5, but P(majority opt)>rt if rt>0.5. This fact is the essence of conformity's power to reduce noise at the individual level into a useful social signal. Conformity works by identifying the optimum disproportionately if other forces, as summarized by rt, bias choices toward the optimum. It does not work, in the sense that it disproportionately identifies the suboptimal technology, if other forces bias choices toward the suboptimum. Moreover, when the social group gets larger, the amount of information embedded in the group increases, and the nonlinearity intrinsic to conformity becomes more extreme. In sum, conformity exaggerates the effectiveness of other decision-making biases such as individual learning in a way that depends on both the size of the social group and the strength of the other bias. If the other bias is bad, conformity is worse. If the other bias is good, conformity is even better. Additionally, this extra bias created by conformity is more extreme for larger social groups.
The Supplementary Material presents additional results formalizing how conformity works. As in the experiment described shortly, the theory focuses on situations in which the payoffs associated with different behaviors are stochastic. The best behavior is not obvious because feedback is noisy. In this case, conformity can filter noisy individual feedback into a powerful signal that points clearly toward the best behavior, but only if some other force is at work.
3. The empirics of frequency dependence
As suggested in the Introduction, conformity should not be defined simply as any positive social influence. Such an approach neglects important distinctions between different types of frequency dependence, some of which produce internally homogeneous groups, and others of which produce social groups that are maximally heterogeneous. Here we outline the distinctions necessary to integrate theory and empiricism into the study of frequency-dependent social learning.
3.1. Individual decisions under fixed and sampled social groups
Boyd & Richerson, 1982, Boyd & Richerson, 1985 developed a simple model of frequency dependence with the following properties. In t+1, each individual in the population samples N individuals from the previous period. Define It∈{0,1,…,N} as a random variable with realizations it specifying the number of individuals choosing R in a particular sample of size N. Given a sample with a particular distribution of behaviors, the response to social information takes the following form for a focal individual,

The parameter D∈[−1,1] controls the nature of frequency dependence. When D∈[−1,0), social learning is nonconformist; when D=0, social learning is linear (Boyd & Richerson, 1985); and when D∈(0,1], it is conformist.
The probabilities specified in Eq. (2) are conditional. They take the distribution of behaviors in the social group (it) as given and do not account for how people form social groups. Conditional probabilities may be relevant in many experimental settings where the social group is fixed (e.g., Asch, 1956), and indeed they will be relevant for the experiments presented below, but they will not always apply. In some cases, we might imagine that individuals estimate the distribution of behaviors in some larger population by sampling behavioral models randomly. In this case, if rt is the frequency of R in the population at t, then the number of individuals who chose R in a sample of individuals will be binomially distributed. In essence, two sources of noise are present. On one hand, given a social group with a particular mix of behaviors, individuals may exhibit positive choice probabilities for both R and B. These are the conditional probabilities of Model 2. On the other hand, if individuals estimate the distribution of behaviors in the population by sampling, samples will typically differ across individuals, and these differences represent another type of noise. Accounting for both types of noise, the unconditional probability under unbiased sampling is simply,

Fig. 2 illustrates the basic features of Models 2 and 3.
Importantly, with sampling, both conformity (Fig. 2C) and nonconformity (Fig. 2D) produce monotonically increasing functions. This means that both are forms of positive social influence. Using basic techniques in nonlinear dynamics (Hoy et al., 2001, McElreath & Boyd, 2007), however, one can show that their aggregate effects are entirely different (Efferson & Richerson, 2007). Conformity produces behavioral homogeneity within the social group or population. In a diametrically opposed fashion, nonconformity produces the maximum amount of heterogeneity.
Without sampling, conformity monotonically increases in the number of individuals exhibiting R (Fig. 2A). Nonconformity monotonically increases over two restricted intervals (Fig. 2B). So long as we restrict attention to rt∈[0,1/2) or rt∈(1/2,1], nonconformity posits, like conformity, that the probability that a focal individual adopts R increases in the number of individuals with behavior R. This assumption is compatible with the idea that individuals show relatively small biases toward the behavior in the minority. These biases are enough to move the group away from the behavior currently in the majority, thus distinguishing nonconformity from conformity, but the biases are not especially large. For this reason, we will refer to this form of nonconformity as “weak” nonconformity. With respect to empirical studies, weak nonconformity has the following important implication. Researchers cannot cleanly identify conformity by simply showing that majorities of different sizes have a positive effect on the rate of adopting the behavior in question (e.g., Asch, 1955). In essence, this is like restricting attention to the interval rt∈(1/2,1], and both conformity and weak nonconformity make the same qualitative prediction over this interval.
In the Supplementary Material, we derive a model of “strong” nonconformity in which individuals exhibit large biases toward the behavior in the current minority. This form of nonconformity produces a model in which the probability of choosing a behavior varies inversely with the behavior's frequency in the population—a qualitative relation very different from any form of Models 2 and 3. Nonetheless, like weak nonconformity and unlike conformity, strong nonconformity cannot produce dynamically stable, behaviorally uniform social groups.
3.2. Only conformity produces internally homogeneous groups
Whether individuals sample or not when forming a group of associates, the critical feature of conformity is that it moves the group toward the behavior in the majority at any given point in time. This is formally captured by the definition presented in the Introduction. In a simple two-behavior setting, if rt<1/2, the probability that a focal individual adopts R in the next period is less than rt. If rt>1/2, the probability is greater than rt. Thus, if R is in the minority, the minority gets progressively smaller until R disappears altogether. If R is in the majority, the majority gets progressively larger until R alone is present.1
Contrast this scenario with the following alternatives. Linear transmission (Boyd & Richerson, 1985) means that the probability that a focal individual chooses R is equal to rt for all values of rt. In this case, the dynamics of behavior are neutral with respect to frequency-dependent social influence. If the distribution of behaviors changes through time, it changes for some other reason and not because people are responding in a biased way to how common behaviors are. Next we consider the two forms of nonconformity. Both assume that if rt<1/2, the probability that a focal individual adopts R in the next period is greater than rt, while if rt>1/2, the probability is less than rt. Consequently, both forms of nonconformity involve a bias away from the behavior currently in the majority. In the case of weak conformity, the bias is relatively small. Dynamically, this means that the group moves smoothly toward a uniform distribution of behaviors, at which point nonconformity becomes irrelevant, and the system stabilizes (Efferson & Richerson, 2007). Strong nonconformity, in contrast, creates oscillations in the sense that the behavior in the majority constantly changes (Supplementary Material). Regardless of whether the dynamics are controlled by weak or strong nonconformity, groups that are stable and homogeneous cannot result. This follows precisely because of the postulated force away from any majority that might be in place at a given point in time.
Importantly, an infinite number of models that do not fall into the categories outlined in this section exist. In particular, we ignore models that meet some of the assumptions of both conformity and nonconformity. Models of this sort imply that some other bias is at work. An example would be a group of individuals who all choose R with a constant probability of .8. This would mean that R is intrinsically more attractive than B for reasons that have nothing to do with R's frequency in the population. We do not mean to imply that such biases do not exist. We think they do, and they probably interact with frequency dependence. But we ignore them here to focus squarely on the theoretical properties of frequency dependence without other matters clouding the issue.
3.3. Asch's study as an illustrative example
Asch, 1955, Asch, 1956 initiated a tradition of conformity research in which the experiment created a conflict between what the experimental subject perceived as true and the opinions of a unanimous majority aligned against her. Unbeknownst to the experimental subject, this unanimous majority with a contradictory opinion was really composed of confederates. A typical setting involves identifying, from a set of lines, the one line that is the same length as some reference line. The experimental subject sees the right answer, but before the subject states her own opinion, the confederates each choose a line that is not the same length as the reference line. The question is whether this information will influence the subject's probability of stating a wrong answer.
Fig. 3 shows the results from one of Asch's experiments in which the size of the social group—and by extension the size of the unanimous majority of confederates—was varied systematically. The social group, including the experimental subject, ranged from 2 to 16, and so here we code this as unanimous majorities ranging in size from 1 to 15. As the independent variable, we show the proportion of the entire group made up of confederates initially proclaiming the wrong answer, which produces variations ranging from 1/2 to 15/16. This coding assumes that, before stating their own answers, the experimental subjects all had an opinion contradictory to the stated answers of the confederates. We do not actually know this, but it is consistent with the error rate in Asch's control sessions without social influence. This type of coding also produces the most variation in the independent variable and is thus the most favorable approach to Asch's study. The response variable is the proportion of experimental subjects who, like the confederates, also stated a wrong answer. Subjects did not sample from a larger population, and conditional choice probabilities are the relevant concept. The data are superimposed on a graph showing the region of probability space compatible with conformity.
![]() |
Fig. 3. Functions lying within the gray area are consistent with conformity. The circles plot data from an experiment in Asch (1955, p. 6) in which the unanimous majority aligned against the experimental subject constituted a proportion of the group ranging from 1/2 to 15/16. The behavior in question is choosing a line of a different length from the reference line. The horizontal axis is the proportion of the group made up of confederates choosing a wrong line. The vertical axis shows the proportion of experimental subjects also choosing a wrong line. Only those data for which Asch provides precise numerical information are shown here. |
We would like to make three points. First, the experimental data lie entirely outside the conformity region. Second, the data only cover the interval from 1/2 to 1. Both conformity and weak nonconformity predict a monotonic increase over this interval, and thus the data are qualitatively consistent with both models. Third, the experimental protocol created a conflict between two different biases: the information provided by the subject's senses and the subject's possible susceptibility to social influence. For this reason, we do not claim, as Fig. 3 might suggest, that Asch (1955, 1956) actually found nonconformity. Rather, we claim that the joint effect of conflicting biases means that we cannot isolate the response to frequency information, and so we cannot conclude that Asch found conformity as we define it, namely a frequency-dependent force that produces homogeneous social groups through time. The key here is the baseline rate of errors. Asch's baseline is the error rate in the absence of social influence, an error rate close to 0. This baseline is indeed interesting and compelling, but it is not the relevant baseline for understanding the dynamical consequences of frequency-dependent social influence. As outlined above, the relevant dynamical baseline is the current distribution of behaviors. Specifically, we need to know how the probability of choosing a behavior in the future compares to the current frequency of the behavior in the group for every conceivable distribution of behaviors. This was the objective of the experimental work to which we now turn.
4. Experimental methods
With 70 students at the University of Zürich and the Swiss Federal Institute of Technology, we conducted the following experiment. In each period, each player faced a choice between one of two technologies (“red” vs. “blue”). Payoffs followed truncated normal distributions, but one color was optimal in that its payoff distribution had a higher expectation. Specifically, payoffs in experimental currency units for the suboptimal technology were distributed N(30,12), and payoffs for the optimum were distributed N(38,12). Both distributions were truncated at 0 and 68, which changed the means and standard deviations slightly, and payoffs were rounded to the nearest integer. Players did not know which color was better, but they could learn through time. The basic experimental problem was thus similar to that in Efferson et al. (2007) and McElreath et al. (2005).
Players made choices for six blocks of 25 periods each. Each block of 25 periods had a randomly selected optimal color, but all players who played together always had the same optimal color. All of this was explained in the instructions before beginning an experimental session. The framing of the choice task was neutral, but players were explicitly told that the more often they chose the optimal color, the more they would earn. In addition, participants viewed an extensive graphical demonstration before the beginning of the experiment. The demonstration produced various animated histograms that gave subjects an intuition for how random payoffs would be generated even if they did not have formal training in probability theory. The entire experiment was conducted on a local computer network using z-Tree (Fischbacher, 2007). The Supplementary Material provides more details.2
Players within a session were divided into two groups that played simultaneously. In one group of five players, each player individually chose one of the two colors in each period and immediately received private information about her realized payoff. These players did not have any information about other players, and so we refer to them as individual learners. In the other group, composed of six to seven players, each player had social information about the distribution of choices (e.g., three red, two blue) among the individual learners. These players did not have any information about their own payoffs, and thus we refer to them as social learners. Social information was available after all individual learners had made their choices in a period but before a given social learner had made her choice. After communicating the social information, each social learner made a choice between the two colors privately and received a payoff. Realized payoffs, however, were never communicated to players in this group, and individual learning was consequently not possible. Social learners, however, did know that players in the associated group of individual learners were receiving individual feedback about payoffs after each of their choices. Immediately after the experiment, all players responded to a brief questionnaire requesting basic sociodemographic information and information about how they made decisions in the experiment. The only payoff information that social learners received was their total earnings after the experiment and questionnaire had been completed.
Subjects were drawn from the extensive subject pool routinely used at the Institute for Empirical Research in Economics in Zürich. Psychology students were excluded. Sessions lasted about 2 h, and payments were made privately after the experiment. The exchange rate was 150 experimental currency units to the Swiss Franc. Total earnings were the sum over all 150 periods. The average payment was 32.68 Swiss Francs (US$25.50 or ˆ20.91).
5. Results and discussion
The value of conformity in this experiment depended on the effectiveness of individual learning. Individual learning was highly effective. Individual learners exhibited a roughly uniform distribution over the two colors in Period 1, but the proportion choosing the optimal color increased dramatically as the 25 periods progressed. Specifically, regressing the proportion of individual learners choosing optimally on period, using the method of Newey and West (1987) to correct for heteroskedasticity and autocorrelation up to Lag 3, produces a highly significant upward trend (p<.01). The estimated coefficient for period is .012, and the R2 value is .930. Thus, on average, the percentage of individual learners choosing optimally in a group of five increased by roughly 30 percentage points over the course of 25 periods.
To test for conformity among social learners, we take Model 2 as our theoretical framework. To evaluate the theory, we estimated the key parameter D using maximum likelihood under three different levels of assumed heterogeneity among social learners (Supplementary Material). The simplest model posits a single value of D over all observations and all social learners. The second model divides social learners into two groups based on their response to a single questionnaire item. Specifically, to have some measure of what subjects thought they were doing in the experiment, the questionnaire asked social learners about their use of social information. They were asked whether they tended to choose (i) the same color as the majority of players in the “other group” (i.e., individual learners); (ii) the same color as the minority of players in the other group; or (iii) neither. Twenty-eight of 40 social learners claimed (i), 11 claimed (iii), and only 1 claimed (ii). Given that only one player claimed to follow the minority, we divided social learners, in an a priori fashion, into those who claimed that they tended to follow the majority during the experiment (Category i) and those who did not (Categories ii and iii). We call these players “stated conformists” and “not stated conformists,” respectively. Our second model estimates a separate D value for each of these two groups. The final model estimates an individual value of D for each social learner (i.e., individual fixed effects). We used AICc, an improved form of Akaike's original criterion (Akaike, 1973, Burnham & Anderson, 2002), as a model-selection criterion. Table 1 summarizes the results.
Table 1.
Model description, the number of estimated parameters, maximized log likelihood (ln L*), Akaike value (AICc), and Akaike weight (wi) for each of the three models fit to the social learners' data |
![]() |
Model | Parameters | ln L* | AICc | wi | ![]() |
---|---|---|---|---|---|---|
![]() |
Single D | 1 | −2159.28 | 4320.56 | 4.11×10−112 | ![]() |
![]() |
Conformist (yes or no) | 2 | −2014.39 | 4032.78 | 1.27×10−49 | ![]() |
![]() |
Fixed effects | 40 | −1863.36 | 3807.60 | >0.99 | ![]() |
Altogether, the experiment produced 5000 observations for social learners, and 3749 of these could be used to estimate D and to calculate AICc values (see Supplementary Material). Akaike weights sum to 1 and summarize the proportional weight of evidence in support of each model, where larger weights indicate more support. The absolute difference between AICc values also has meaning (Burnham & Anderson, 2002) and is the basis for our claim that the two-parameter model is a vast improvement over the one-parameter model. |
The model of individual fixed effects fits the best to an overwhelming degree, but the AICc values also indicate that the two-parameter model is a vast improvement over the simple model that estimates a single D value. In particular, lower AICc values indicate an improved fit, and the absolute difference in the AICc values between models has meaning. Importantly, however, the use of information-theoretic criteria such as AICc does not involve arbitrary thresholds (e.g., p≤.05) as in hypothesis testing, so there is no concept of one model being “significantly” better than another. But the differences in Table 1 are truly enormous by any standard (Burnham & Anderson, 2002). This finding means that individual variation in frequency-dependent social learning is extremely important, but nonetheless the distinction between stated conformists and those who were not stated conformists also captures an important systematic variation relative to a model that simply assumes that all social learners were the same.
Fig. 4 compares the data and the two-parameter version of the model.
The model fits poorly for social learners who were not stated conformists, but fits quite well for those who were stated conformists. The 12 social learners who were not stated conformists, in effect, did not respond, on average, to information about the frequencies of alternative behaviors in any notable way. Thus, the model, although it can be fit using maximum likelihood, is not based on assumptions that were generally appropriate for social learners in this group. For the 28 stated conformists, however, the data and the model are nearly indistinguishable for much of the function's domain. The exceptions at the boundaries show that stated conformists had a small tendency to play the absent color when all five individual learners were choosing the same color.
Fig. 5 additionally shows considerable individual variation in play among both stated conformists and social learners who were not stated conformists. The graph shows results from the fixed-effects model and plots the mean earnings per period for each subject as a function of the subject's individual D estimate.
Importantly, the individual D estimates are not obviously clustered into two groups. This is why the model of fixed effects fits better than the model that distinguishes simply between stated conformists and everyone else. Additionally, Fig. 5 also clarifies why the distinction between stated conformists and those who were not stated conformists is better than assuming a homogeneous response to frequency information. Most of the stated conformists have positive D estimates under the fixed-effects model, while most of those who were not stated conformists have negative D estimates. In short, players were pretty good, although not perfect, at summarizing their use of frequency information. The net consequence of these results is the following. Fig. 5 provides no obvious evidence for different categorical types of social learners. Nonetheless, when forced to categorize their use of frequency information, social learners did so with enough self-awareness that their claims about their use of frequency information were sufficiently accurate to provide an effective partition of the data. For these reasons, our statistical models fit progressively better as we added parameters. By adding parameters, we increasingly captured considerable variation among social learners.
Finally, Fig. 5 shows, as predicted by theory (Model 1), a strong positive relationship between conformity and earnings. In short, because the individual learners were actually learning, social learners who showed a strong inclination to follow the majority among individual learners were the social learners who made the most money. Social learners who did not respond to the available frequency-dependent information left money on the table.
These results show that individual heterogeneity is critical to understanding frequency-dependent social learning. Specifically, our data suggest a meaningful distinction between those who conform and those who largely ignore information about behavioral frequencies. Nonetheless, substantial individual variation also exists within each of these two generic groups of players. The stability of this kind of heterogeneity across cultures or in different social settings is not clear, but an analogous study recently conducted among subsistence pastoralists in Bolivia produced similar patterns (Efferson, Lalive, Richerson, McElreath, & Lubell, unpublished data). Also unclear is the extent to which players might adjust their use of frequency-dependent social information according to its value. Do some players, for example, have an innate desire to conform regardless of the consequences, or do they rather recognize its practical decision-making value in appropriate situations? Our results show that some individuals do not conform even when doing so would be very much in their own interests. This conclusion is in contrast to studies such as those of Asch, 1955, Asch, 1956, which tends to focus on how some will cave in to social influence even in direct opposition to what their senses are telling them.
Apart from the subjects who largely ignored frequency information, many did conform, and doing so paid well. To fully examine in the future how subjects adjust their tendency to follow the majority, its value would have to be systematically and exhaustively varied. The present experiment did not do so. Because the value of conformity was rooted in the performance of individual learners, conformity, on average, was either neutral (e.g., Period 1) or valuable (e.g., subsequent periods). It was never detrimental on average. Thus, we cannot say to what extent social learners who conformed recognized conformity's monetary value in this particular experiment and to what extent they simply had a desire to match the properties of the social group provided for them regardless of the monetary consequences. More generally, the issue of how flexible biased social learning is in different settings and over short time scales remains one of the central unanswered empirical questions in the study of cultural transmission.
The heterogeneity in social learning that we have documented has received little attention in the study of cultural transmission. In particular, if distinct and stable types of social learners exist, one obviously important consideration would involve how they assort into groups both within and between societies. For instance, if all else is equal and conformists form groups assortatively, conformist groups should be more productive than their less conformist counterparts as long as some basis for effective individual learning is present. More generally, the study of dynamical systems can sometimes proceed effectively by focusing on the average behavior of constituent parts, while in other cases ignoring individual variation can lead the researcher dramatically astray (Miller & Page, 2007). Which of these two scenarios holds and when are largely unconsidered problems in cultural evolution. Even so, this kind of understanding is potentially critical when addressing aggregate behavioral dynamics and the corresponding evolutionary consequences for organisms with biased cultural transmission.
Acknowledgments
The authors would like to thank all the members in the chair of Ernst Fehr at the Institute for Empirical Research in Economics for assistance and comments. Particular thanks go to Urs Fischbacher for his z-Tree program, which was used to produce animated histograms. We would also like to acknowledge two anonymous reviewers for much helpful input.
References
Akaike, 1973 1.Information theory as an extension of the maximum likelihood principle. . In: Petrov BN, Csaki F editor. Second international symposium on information theory. Budapest: Akademiai Kiado; 1973;p. 267–281.
Aronson et al., 2002 2.Social psychology. . 4th ed.. Upper Saddle River, NJ: Prentice-Hall; 2002;.
Asch, 1955 3.Opinions and social pressure. . Scientific American. 1955;193(5):1–7.
Asch, 1956 4.Studies of independence and conformity: I. A minority of one against a unanimous majority. . Psychological Monographs. 1956;70:1–70.
Berns et al., 2005 5.Neurobiological correlates of social conformity and independence during mental rotation. . Biological Psychiatry. 2005;58(3):245–253. Abstract | Full Text | Full-Text PDF (460 KB) | MEDLINE | CrossRef
Bowles, 2004 6.Microeconomics: Behavior, institutions, and evolution. . New York: Russell Sage; 2004;.
Boyd et al., 2003 7.The evolution of altruistic punishment. . Proceedings of the National Academy of Sciences. 2003;100(6):3531–3535.
Boyd & Richerson, 1982 8.Cultural transmission and the evolution of cooperative behavior. . Human Ecology. 1982;10(3):325–351.
Boyd & Richerson, 1985 9.Culture and the evolutionary process. . Chicago: University of Chicago Press; 1985;.
Boyd & Richerson, 2005 10.The origin and evolution of cultures. . Oxford: Oxford University Press; 2005;.
Burnham & Anderson, 2002 11.Model selection and multimodel inference: A practical information-theoretic approach. . 2nd ed.. New York: Springer-Verlag; 2002;.
Efferson & Richerson, 2007 12.A prolegomenon to nonlinear empiricism in the human behavioral sciences. . Biology and Philosophy. 2007;22(1):1–33.
Efferson et al., 2007 13.Learning, productivity, and noise: An experimental study of cultural transmission on the Bolivian altiplano. . Evolution and Human Behavior. 2007;28(1):11–17.
Fehr & Fischbacher, 2003 14.The nature of human altruism. . Nature. 2003;425:785–791. CrossRef
Fehr & Fischbacher, 2004 15.Social norms and human cooperation. . Trends in Cognitive Sciences. 2004;8(4):185–190. MEDLINE | CrossRef
Fehr & Gaechter, 2002 16.Altruistic punishment in humans. . Nature. 2002;415:137–140. MEDLINE | CrossRef
Fischbacher, 2007 17.z-Tree: Zurich toolbox for ready-made economic experiments. . Experimental Economics. 2007;10(2):171–178.
Guererk et al., 2006 18.The competitive advantage of sanctioning institutions. . Science. 2006;312:108–111. CrossRef
Guzmán et al., 2006 19.When in Rome, do as the Romans do: The coevolution of altruistic punishment, conformist learning, and cooperation. . Evolution and Human Behavior. 2007;28:112–117.
Henrich, 2001 20.Cultural transmission and the diffusion of innovations: Adoption dynamics indicate that biased cultural transmission is the predominate force in behavioral change. . American Anthropologist. 2001;103(4):992–1013.
Henrich, 2004 21.Cultural group selection, coevolutionary processes and large-scale cooperation. . Journal of Economic Behavior and Organization. 2004;53(1):3–35.
Henrich & Boyd, 1998 22.The evolution of conformist transmission and the emergence of between-group differences. . Evolution and Human Behavior. 1998;19(4):215–241.
Henrich & Boyd, 2001 23.Why punish defectors: Weak conformist transmission can stabilize costly enforcement of norms in cooperative dilemmas. . Journal of Theoretical Biology. 2001;208(1):79–89. MEDLINE | CrossRef
Henrich & McElreath, 2003 24.The evolution of cultural evolution. . Evolutionary Anthropology. 2003;12(3):123–135.
Hoy et al., 2001 25.Mathematics for economics. . 2nd ed.. Cambridge: The MIT Press; 2001;.
Lumsden & Wilson, 1980 26.Translation of epigenetic rules of individual behavior into ethnographic patterns. . Proceedings of the National Academy of Sciences. 1980;77(7):4382–4386.
McElreath & Boyd, 2007 27.Mathematical models of social evolution: A guide for the perplexed. . Chicago: University of Chicago Press; 2007;.
McElreath et al., 2005 28.Applying evolutionary models to the laboratory study of social learning. . Evolution and Human Behavior. 2005;26(6):483–508.
Miller & Page, 2007 29.Complex adaptive systems: An introduction to computational models of social life. . Princeton: Princeton University Press; 2007;.
Newey & West, 1987 30.A simple, positive semi-definite, heteroskedasticity and autocorrelation consistent covariance matrix. . Econometrica. 1987;55(3):703–708.
Richerson & Boyd, 2005 31.Not by genes alone: How culture transformed the evolutionary process. . Chicago: University of Chicago Press; 2005;.
Rogers, 1995 32.Diffusion of innovations. . 4th ed.. New York: The Free Press; 1995;.
Sherif & Murphy, 1936 33.The psychology of social norms. . New York: Harper and Brothers; 1936;.
Whiten et al., 2005 34.Conformity to cultural norms of tool use in chimpanzees. . Nature. 2005;437:737–740. CrossRef
a Santa Fe Institute, Santa Fe, NM 87501, USA
b Institute for Empirical Research in Economics, University of Zürich, 8006 Zürich, Switzerland
c Department of Econometrics and Political Economy, University of Lausanne, 1015 Lausanne, Switzerland
d Graduate Group in Ecology, University of California, Davis, Davis, CA 95616, USA
e Environmental Science and Policy, University of California, Davis, Davis, CA, USA
f Anthropology, University of California, Davis, Davis, CA, USA
Corresponding author. Santa Fe Institute, Santa Fe, NM 87501, USA.
☆ C.E. thanks the US Environmental Protection Agency (STAR fellowship no. U-91615601-3) for financial support. This research was funded by a US National Science Foundation grant (award no. 0340148 to P.J.R., R.M., and M.L.).
1 Strictly speaking, this claim about perfect homogeneity requires that the function specifying choice probabilities includes the points (0,0) and (1,1) and is continuous in the vicinity of both points. Otherwise, the result is weaker. The group is increasingly homogeneous, but perfect homogeneity does not result.
2 Instructions in German and/or z-Tree code is available upon request.
PII: S1090-5138(07)00087-6
doi:10.1016/j.evolhumbehav.2007.08.003
© 2008 Elsevier Inc. All rights reserved.